Machine Learning Conference for X-Ray and Neutron-Based Experiments, Munich 2024
Bürgerhaus 1 - Bürgerhaus Main ball room
Bürgerhaus Garching
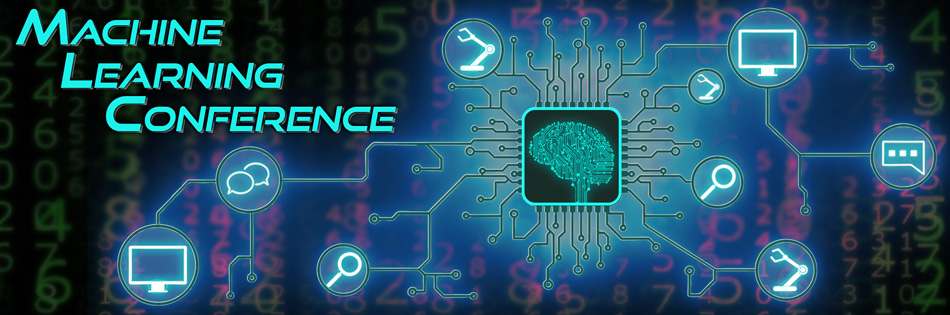
From April 8 to 10, 2024 the Heinz Maier-Leibnitz Zentrum (MLZ) located in Garching near Munich will host the Machine Learning Conference for X-Ray and Neutron-Based Experiments.
The conference is a follow-up of the Machine Learning Workshop for X-ray and Neutron Scattering at Lawrence Berkeley National Laboratory in April 2023.
The application of machine learning (ML) tools for data generated at photon and neutron (PaN) large scale facilities offers new opportunities and challenges. This conference will gather experts from the field of machine learning as well as specialists for the application of neutron and photon beams to discuss their latest research. Discussion topics will range from autonomous experiment control, data analysis tools to requirements for ML training data. In addition to presentations, the event will also include demonstrations of ML software and opportunities for attendees to ask questions and discuss future directions.
The first two days (April 8/9) will take place at the Community Center (Bürgerhaus) Garching with all participants. The third day (April 10) offers optional workshops and hands-on tutorials as well as reactor tours at the site of research neutron source FRM II for all interested participants.
We are looking forward to seeing you in Garching!
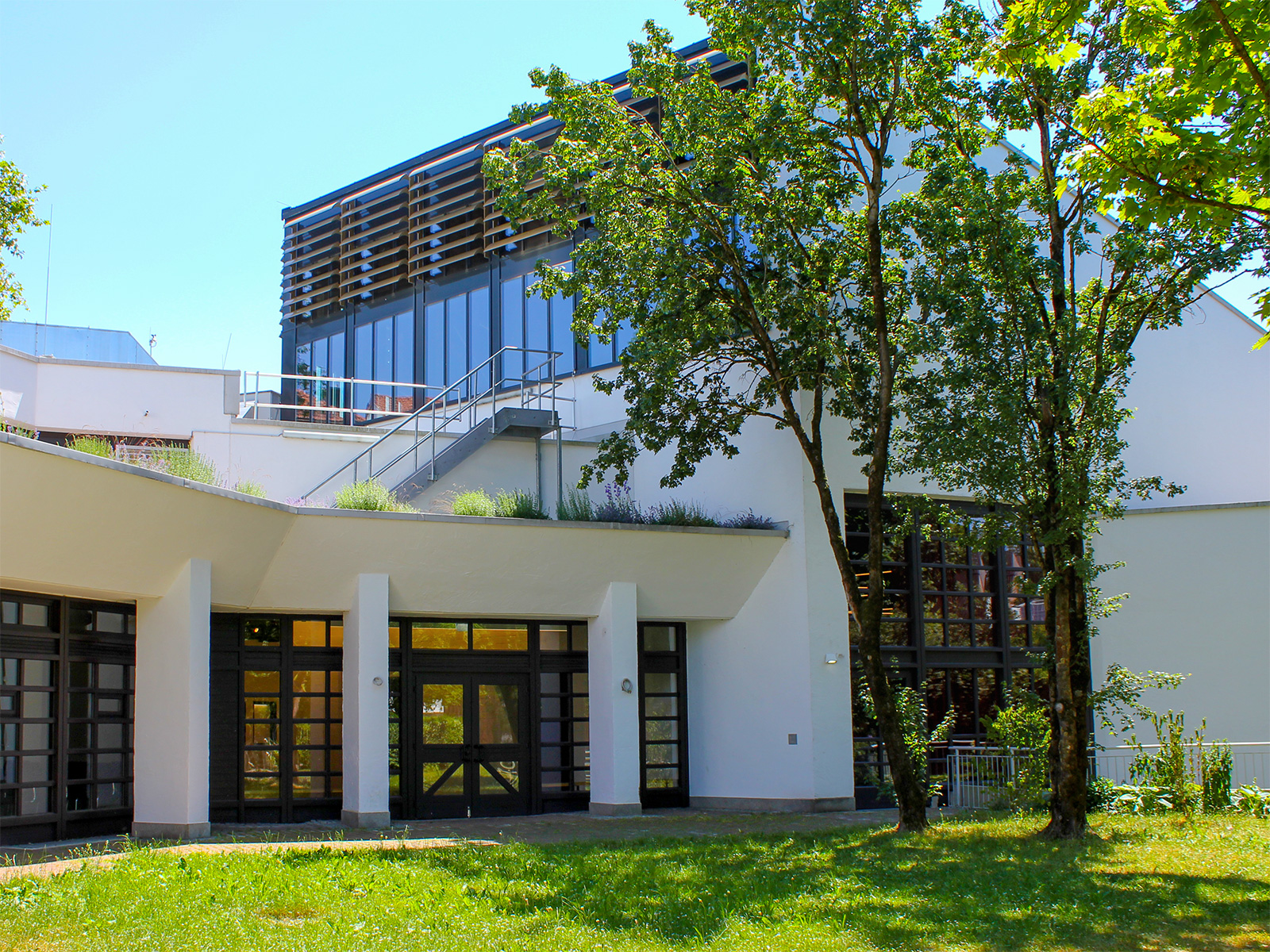
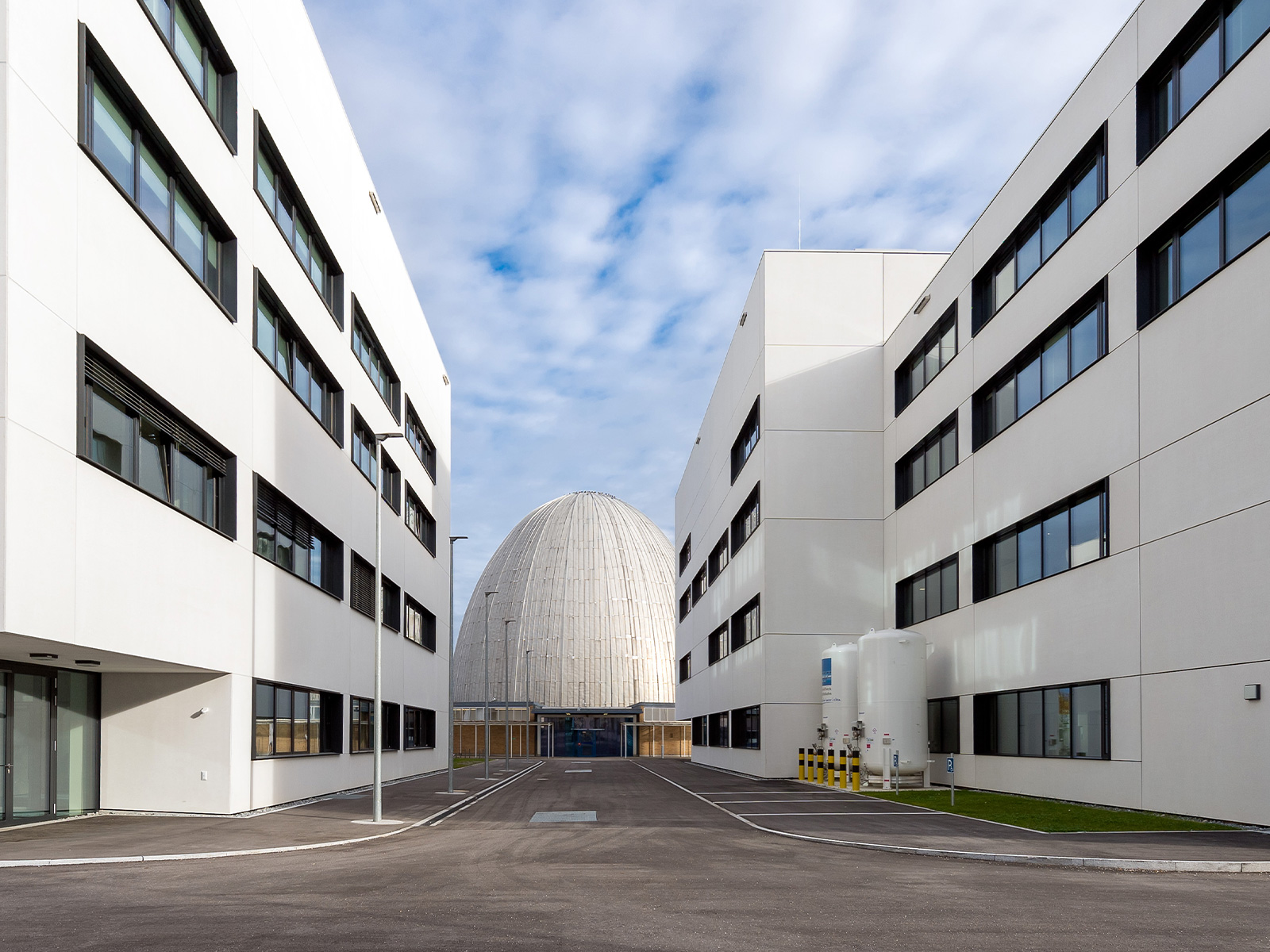
The two venues for the conference: On the left, Garching Community Center (Bürgerhaus) which is the conference location on April 8 and 9. On the right, FRM II Site Garching which hosts optional hands-on workshops and reactor tours on April 10.
Header logo: © FRM II / TUM
Photo bottom left: © FRM II / TUM
Photo bottom right: © Astrid Eckert
-
-
08:00
→
09:00
Registration Bürgerhaus 1 - Bürgerhaus Main ball room
Bürgerhaus 1 - Bürgerhaus Main ball room
Bürgerhaus Garching
Bürgerplatz 9 and Telschowstraße 4, 85748 Garching bei München and MLZ Lichtenbergstr. 1 85747 Garching200Show room on map -
09:00
→
09:20
Opening Remarks
-
09:20
→
10:30
Session 1 Bürgerhaus 1 - Bürgerhaus Main ball room
Bürgerhaus 1 - Bürgerhaus Main ball room
Bürgerhaus Garching
Bürgerplatz 9 and Telschowstraße 4, 85748 Garching bei München and MLZ Lichtenbergstr. 1 85747 Garching200Show room on map-
09:20
Deep learning-based feature detection on 2D X-ray surface scattering data 30m
Grazing-incidence Wide Angle X-ray scattering (GIWAXS) is a key technique for characterizing surface structures of thin films. The method can be used for in-situ experiments monitoring growth and crystallization effects in real-time, but it produces large amounts of data, frequently exceeding the capabilities of traditional data processing methods.
Feature detection in multidimensional X-ray data poses a critical challenge in automated analysis. Especially, in datasets with low signal-to-noise ratio and experimental artifacts in the data, classical peak finding methods may fail.
We demonstrate an automated pipeline for the analysis of GIWAXS images, based on the Faster Region-based Convolutional Network architecture for object detection, modified to conform to the specifics of GIWAXS data. The model exhibits high accuracy in detecting diffraction features on noisy patterns with various experimental artifacts. We demonstrate our method on real-time tracking of organic-inorganic perovskite crystallization.
We will discuss the performance of the ML peak finding approach in comparison to classical peak detection methods on the basis of a manually annotated dataset of 35 GIWAXS images.V. Starostin et al., npj Comput Mater 8 (2022) 101
V. Starostin et al., Synchrotron Radiation News 35 (2022) 21
A. Hinderhofer et al., J. Appl. Cryst. (2023). 56, 3-11Speaker: Alexander Hinderhofer (University of Tübingen) -
09:50
Adaptive X-Ray Reflectivity Measurements using Deep Reinforcement Learning 20m
This presentation focuses on the application of machine learning techniques, specifically deep reinforcement learning, to improve the process of X-ray reflectivity (XRR) measurements. Our study demonstrates how machine learning can be utilized to dynamically adjust measurement angles and integration times, adapting these parameters after acquisition of each new datapoint to optimize the information gathered about the sample.
In our approach, a diffractometer is simulated within a virtual environment including the simulation of the scattering process but also including motor movement times. Here, a machine learning agent is trained to select data points in a smart and adaptive manner, using information from previous simulated measurements to inform its decisions. The agent is designed to prioritize the speed of measurements and accuracy, receiving rewards for achieving quicker measurements and reducing the error in predicting the layer parameters from the measured curve. Once trained, this agent then is used to steer a real laboratory diffractometer via the BLISS (ESRF) control software. The key outcome of this research is a significant increase in measurement efficiency. Our findings indicate that using this machine learning approach one can easily speed up the measurement process by at least a factor of two, and also reduce measurement errors. The “self-driving diffractometer” using a reinforcement learning agent presents an advancement not only for XRR measurements, but the results also apply to many X-ray and neutron scattering experiments using angular scans.Speaker: Prof. Stefan Kowarik (Universität Graz) -
10:10
Tracking morphological changes during sputter deposition using GISAXS and machine learning 20m
Solving inverse problems is the basis of the analysis of scattering experiments. The difficulty stems from the fact that the real-space structure has to be retrieved from reciprocal space information. With respect to thin films and interfaces, grazing incidence small- angle X-ray scattering (GISAXS) is a powerful tool for accessing their nanoscale structure formation. GISAXS allows for experiment in real time with high time resolution and high statistical relevance[1]. The two-dimensional scattering pattern is governed by the distorted-wave Born approximation (DWBA) - refraction and reflection effects have to be considered, adding further to complexity in data analysis. Hence, a model-based approach using simulations for the GISAXS pattern is necessary for elucidating the nanostructure[2,3].
Sputter deposition is an industrially-relevant method for fabricating metal-polymer nanocomposites[3]. The time resolution down to the sub-millisecond scale combined with in situ sputter deposition yields a large amount of data that requires careful analysis[4]. One way to extract quantitative information is to use a data base of model simulations of the sample. While fundamental assumptions about the system must be made in order to establish the simulations[5], the choice of appropriate inputs leads to a good approximation of the GISAXS data. A key issue is finding the simulation that best represents the system at each stage of the experiment.
Neural networks (NNs) are used to predict the behavior of a system through mathematical modeling. In our case, we use as preprocessing a background and intensity thresholding following Parente et al. [6] with the thresholding factor β being the only variable in the preprocessing stage. Additionally, we tested different network architectures using non-linear activations functions ReLU (R) and Leaky ReLU (L) in different compositions. We present the results of a multilayer perceptron and a convolutional NN (CNN) concerning the structure and morphology of the cluster growth of gold in silicon during sputter deposition. Especially the prediction of the percolation threshold is discussed.
[1] S. Liang, M. Schwartzkopf, S. V. Roth, P. Müller-Buschbaum, Nanoscale Adv. 2022, 4, 2533.
[2] Q. Chen, C. J. Brett, A. Chumakov, M. Gensch, M. Schwartzkopf, V. Körstgens, L. D. Söderberg, A. Plech, P. Zhang, P. Müller-Buschbaum, S. V Roth, ACS Appl. Nano Mater. 2021, 4, 503.
[3] S. V Roth, H. Walter, M. Burghammer, C. Riekel, B. Lengeler, C. Schroer, M. Kuhlmann, T. Walther, A. Sehrbrock, R. Domnick, P. Müller-Buschbaum, Appl. Phys. Lett. 2006, 88, 021910.
[4] M. Schwartzkopf, A. Hinz, O. Polonskyi, T. Strunskus, F. C. Löhrer, V. Körstgens, P. Müller-Buschbaum, F. Faupel, S. V. Roth, ACS Appl. Mater. Interfaces 2017, 9, 5629.
[5] M. Schwartzkopf, A. Buffet, V. Körstgens, E. Metwalli, K. Schlage, G. Benecke, J. Perlich, M. Rawolle, A. Rothkirch, B. Heidmann, G. Herzog, P. Müller-Buschbaum, R. Röhlsberger, R. Gehrke, N. Stribeck, S. V Roth, Nanoscale 2013, 5, 5053.
[6] M. Teixeira Parente, G. Brandl, C. Franz, U. Stuhr, M. Ganeva, A. Schneidewind, Nat. Commun. 2023, 14, 2246.Speaker: Stephan Roth (DESY / KTH)
-
09:20
-
10:30
→
11:00
Coffee Break 30m Bürgerhaus 1 - Bürgerhaus Main ball room
Bürgerhaus 1 - Bürgerhaus Main ball room
Bürgerhaus Garching
Bürgerplatz 9 and Telschowstraße 4, 85748 Garching bei München and MLZ Lichtenbergstr. 1 85747 Garching200Show room on map -
11:00
→
13:00
Session 2 Bürgerhaus 1 - Bürgerhaus Main ball room
Bürgerhaus 1 - Bürgerhaus Main ball room
Bürgerhaus Garching
Bürgerplatz 9 and Telschowstraße 4, 85748 Garching bei München and MLZ Lichtenbergstr. 1 85747 Garching200Show room on map-
11:00
Characterizing quantum materials with scattering experiments and machine learning 30m
The past few years have witnessed booming research in machine learning in chemistry and materials sciences. New pharmaceutical molecules and new energy materials have been identified by machine learning, leading to a paradigm shift in research and industry. Quantum materials, on the other hand, despite constant new reports in using machine learning, have experienced significant challenge due to the complex interplay between the charge, spin, orbital, and lattice degrees of freedom, and the often-met out-of-distribution (OOD) problem.
In this talk, we introduce our recent efforts in connecting machine learning to various quantum materials with various experimental scattering and spectroscopic techniques. For topological materials with band topology, since “topology” itself is not measurable, seeking the experimental manifestation becomes critical. We introduce our recent effort, to use machine learning to improve neutron measurement resolution [1], and to use x-ray absorption to detect topology with 90% accuracy [2]. For collective excitations of phonons, measurable by inelastic scattering, we show how 3D symmetry can be encoded into a neural network that could lead to efficient property predictions [3], and beyond that how to encode the Brillouin zone into real-space graph neural network to predict complex materials [4]. We present our most recent work on machine learning to classify Majorana bound state from tunneling conductance data, even the OOD problem is severe [5]. We conclude by presenting a few more examples showing the increasingly important role machine learning may play in a variety of quantum many-body and scattering experiments even with scarcity of data and challenges in computation.
[1] NA, ZC, ML, Appl. Phys. Rev. 9, 011421 (2022)
[2] NA, ML Advanced Materials 34, 202204113 (2022)
[3] ZC, NA, ML. Adv. Sci. 8, 2004214 (2021), ZC, XS, ML, Advanced Materials 35, 2206997 (2023)
[4] RO, AC, ML, arXiv:2301.02197 (2023).
[5] MC, RO, AC, ML, arXiv:2310.18439 (2023).Speaker: Mingda Li (Massachusetts Institute of Technology (MIT)) -
11:30
Semi-supervised approaches to determining space groups and Bravais Lattices 20m
During this talk, I will discuss our work [1] to use neural networks to automatically classifiy Bravais lattices and space-groups from
neutron powder diffraction data. Our work classifies 14 Bravais lattices and 144 space groups. The novelty of our approach is to use semi- supervised and self-supervised learning to allow for training on data sets with unlabeled data as is common at user facilities. We achieve state of the art results with a semi-supervised approach. Our accuracy for our self-supervised training is comparable to that with a supervised approach. *Support for Satvik Lolla was provided by the Center for High-Resolution Neutron Scattering, a partnership between the National Institute of Standards and Technology and the National Science Foundation under Agreement No. DMR-2010792Speaker: Dr William Ratcliff (National Institute of Standards and Technology) -
11:50
Machine learning assisted automation of single crystal neutron diffraction using ReTIA 20m
Neutron scattering is a versatile and powerful technique widely used in materials science to gain insights into materials' properties and uncover new materials. However, this method is often expensive and time-consuming, requiring advanced detector technology and complex data reduction and analysis procedures. Machine learning (ML) has opened new avenues for neutron diffraction data reduction and experiment operation. In this regard, an ML-assisted real-time image analysis method (ReTIA) has been proposed and utilized at HB-3A DEMAND at HFIR, ORNL. The method is designed to recognize Bragg peaks with high precision and identify the corresponding regions of interest. Once the peaks are recognized, the method can automatically align a measured crystal and optimize the data collection using user-provided information and uncertainty quantification values of the detected peaks. The proposed method has been shown to perform exceptionally well in various complex sample environments, enabling automated single-crystal neutron diffraction. This method's success can dramatically accelerate the discovery of new materials with unique properties. The ability to automate the data collection process will also free up valuable time for researchers to focus on other aspects of their research, leading to more efficient and productive experiments.
The research at Oak Ridge National Laboratory (ORNL) was supported by the U.S. Department of Energy (DOE), Office of Science, Office of Advanced Scientific Computing Research under the contract ERKJ387, and Office of Basic Energy Sciences, Early Career Research Program Award KC0402020, under Contract DE-AC05-00OR22725. This research used resources at the High Flux Isotope Reactor, a DOE Office of Science User Facility operated by ORNL.
Speaker: Huibo Cao (Neutron Scattering Division, Oak Ridge National Laboratory) -
12:10
Combined phase contrast imaging and diffraction at extreme conditions for determination of kinetic parameters 20m
Very recently, it became possible to combine propagation-based phase contrast-imaging (PCI) and X-ray diffraction at extreme conditions at the Extreme Conditions Beamline (P02.2), PETRA III, DESY, Hamburg. This first platform for such experiments enables the investigation of hierarchical structures at conditions approaching those observed in the internal structure of planets, with pressures exceeding 10,000 atm. In PCI, the incident X-ray wave front gets modified by the complex refractive index of the material and the propagation provides the phase information as measurable intensities. The partially coherent X-ray beam produces edge-enhancement in PCI, which provides strong contrast at phase boundaries revealing information on crystallization, solid-solid phase transitions and melting. Therefore, PCI can overcome the complications with absorption-based techniques, which are not useful for low-Z materials and for materials with small density differences. A successful flat-field correction is achieved by computing the principal component analysis (PCA) components on all images and finally to obtain the image in the object plane, phase retrieval algorithms are necessary.
This project concerns how the PCI can be used to extract information about kinetics of a sample system through the observation of micrometer-sized features, such as grain size. Meanwhile, X-ray diffraction is used as a secondary probe for sub-nanometer identification of the structural properties. The extreme states of matter are replicated using so-called diamond anvil cells (DAC) for pressure generation, which can be combined with infrared lasers to generate temperatures larger than 2,000 K. One further development of the DAC is the dynamic DAC, where, by applying a voltage profile to a piezo, the compression rate can be varied up to roughly 100 TPa/s. This enables the investigation of material properties – and kinetics – across a multitude of orders in magnitude in the compression rate.
Here, we are interested in simple systems, like gallium, pure water, and platinum, to test the capabilities of combined PCI with X-ray diffraction. We will describe our trials using standard segmentation analysis methods such as Chan-Vese segmentation, edge-detection and watershed, with that of the manual approach as well as a machine-learning approach and finally show that good agreement can be found between manually determining the size of grains and that found from segmentation using machine-learning. We will end with an outlook critically discussing the effectiveness of the machine-learning algorithms during data analysis and propose new ways of incorporating machine-learning into more parts of the project ultimately leading towards becoming a part of the beamline operation.Speaker: Emma Ehrenreich-Petersen (Deutsches Elektronen-Synchrotron DESY) -
12:30
Fast and reliable crystal structure prediction using random search algorithms and machine learning interatomic potentials 20m
The position that ions occupy in the unit cell of a crystal and in the periodic table of elements, fully determines the physical, chemical and functional properties of materials. Through diffraction experiments, such as X-ray and neutron scattering, it is possible to determine the crystal structure of a material. However, when such experiments are difficult to conduct (e.g. requiring high-pressure conditions) and in situations in which rational guides are required to avoid expensive and time-consuming trial-error searches (e.g. design of new drugs), conducting initial crystal structure prediction (CSP) studies may provide useful insights. This is a formidable complicated problem, since stable and meta-stable phases are related with minima in the energy hyper-surface of the configuration space, and to explore this surface is a tremendously complicated task [1]. Different methodologies of CSP have been developed and proven useful in various situations [2]. Here we present PyMCSP [3] (Python and Machine Learning methods implementation for Crystal Structure Prediction). The program uses random search methodologies to look for the energy minima, by using the PyXtal library [4] to generate different phases with different symmetries compatible with the stoichiometry of the material. Afterwards, the structures are relaxed using machine learning interatomic potentials with M3GNet [5], and ranked according to their energy. By proceeding in this manner, we avoid the use of computationally intensive first-principles calculations (e.g. density functional theory, DFT) and supercomputer facilities so that full CSP analysis can be carried out in very short timeframes using only a laptop. It is possible to determine a theoretical diffractogram of the resulting phases and compare it to those obtained experimentally, in order to assist with space group identification. The reliability of the results has been tested in different materials, for example obtaining some of the meta-stable phases of the polymorphic materials BN or Ag3SBr.
[1] Artem R Oganov, Chris J Pickard, Qiang Zhu, and Richard J Needs. Structure prediction drives materials discovery. Nature Reviews Materials, 4(5):331–348, 2019.
[2] Scott M Woodley and Richard Catlow. Crystal structure prediction from first principles. Nature materials, 7(12):937–946, 2008.
[3] https://github.com/polbeni/PyMCSP.
[4] Scott Fredericks, Kevin Parrish, Dean Sayre, and Qiang Zhu. PyXtal: A Python library for crystal structure generation and symmetry analysis. Computer Physics Communications, 261:107810, 2021.
[5] Chi Chen and Shyue Ping Ong. A universal graph deep learning interatomic potential for the periodic table. Nature Computational Science, 2(11):718–728, 2022.Speaker: Pol Benítez Colominas (Universitat Politècnica de Catalunya)
-
11:00
-
13:00
→
14:30
Lunch Break 1h 30m Bürgerhaus 1 - Bürgerhaus Main ball room
Bürgerhaus 1 - Bürgerhaus Main ball room
Bürgerhaus Garching
Bürgerplatz 9 and Telschowstraße 4, 85748 Garching bei München and MLZ Lichtenbergstr. 1 85747 Garching200Show room on map -
14:30
→
16:20
Session 3 Bürgerhaus 1 - Bürgerhaus Main ball room
Bürgerhaus 1 - Bürgerhaus Main ball room
Bürgerhaus Garching
Bürgerplatz 9 and Telschowstraße 4, 85748 Garching bei München and MLZ Lichtenbergstr. 1 85747 Garching200Show room on map-
14:30
Generative machine learning for scattering and spectroscopy data analysis 30m
Supervised machine learning (ML) models are frequently trained on large datasets of physics-based simulations with the aim of being applied for experimental scattering or spectroscopy data analysis. However, ML models trained on simulated data often struggle to perform on experimental data. Two primary challenges are handling data from structures not present in the training database and accounting for experimental data that contains signals not included in the simulated data.
Generative ML can be used to address both challenges by learning the underlying distribution of the data. I will discuss how we use generative ML to solve mono-metallic nanoparticles given pair distribution function data previously unseen by the model and how generative ML can be used to convert a simulated inelastic neutron scattering dataset into one that resembles an experiment, and vice versa.
Speaker: Andy Sode Anker (Department of Energy, Danish Technical University, Denmark) -
15:00
Equivariant Generative Models: Atomic-Scale Representations from Diffraction Patterns 20m
Keywords: Materials Characterization, Diffraction Techniques, Machine Learning, Probabilistic Models, Structural Analysis.
Understanding a material inexorably requires from the determination of its atomic structure by means of neutron and x-rays based diffraction techniques. However, although artificial intelligence has shown valuable help in property-prediction lately [1], previous machine learning characterizations of diffraction patterns often yield vague insights. This work introduces a groundbreaking framework built upon denoising probabilistic diffusion models, such as those in which ChatGPT or DALL-E are based [2, 3], offering a transformative approach to extracting realistic atomistic snapshots of materials directly from their diffractograms.
The proposed framework would transcend the limitations of conventional methods by not only discerning atomic species but also precisely determining their positions within the material. Remarkably, it would extend its capability to identify potential secondary phases present in the material, leveraging the rich structural information embedded in diffractograms.
To enhance the performance of the model, our approach allows the incorporation of a priori knowledge about the expected presence or absence of specific atoms. This feature is particularly beneficial when a high level of certainty exists regarding chemical composition.
In our ongoing research, we are applying this innovative methodology to the emerging class of chalcohalide-based solar cell absorbers, to which our experimental group is devoted. The wealth of x-ray diffraction patterns available for these materials serves as a robust testing ground. The results obtained thus far underscore the potential of our framework to provide unprecedented clarity on the atomic-scale structure of complex materials.
[1] C. López, A. Emperador, E. Saucedo, et al. Universal ion-transport descriptors and classes of inorganic solid-state electrolytes, Materials Horizons, 2023.
[2] OpenAI (2023) ChatGPT
[3] OpenAI (2023) DALL-ESpeaker: Cibrán López Álvarez (Polytechnic University of Catalonia) -
15:20
Solving atomic structures by combining analysis of PDF pattern with DFT calculations in machine learning enhanced global optimization 20m
Determination of crystal structures of nano-crystalline, or amorphous compounds is a great challenge in solid states chemistry and physics. Structural analysis using atomic pair distribution function (PDF) of X-ray or neutron total scattering data has the potential to become a very efficient method in this field. Unfortunately, for real-space structure refinements using this method, an initial starting model for the atomic positions is needed, but not available in many cases. To solve this problem, we have recently introduced an algorithm [1, 2] that is able to determine the crystal structure of an unknown compound by means of an on-the-fly trained machine learning (ML) model that combines density functional calculations (DFT) with comparison of calculated and measured PDFs for global optimization. The PDF might be obtained from X-Ray or neutron scattering Data or a combination of both. In our previous work, we showed, that the algorithm is able to predict stacking disorder in layered compounds and even meta-stable point defects in spinel structures with particle sizes below 4 nm. In an ongoing study, we are focusing on even smaller particle sizes and are now able to present all-atom structure models of supported and unsupported particles down to the 1 nm range.
[1] M. Kløve, S. Sommer, B.B. Iversen, B. Hammer, W. Dononelli; Machine learning based approach for solving atomic structures of nanomaterials combining pair distribution functions with density functional theory, Advanced Materials 35, 2208220 (2023)
[2] W. Dononelli; Das Experiment direkt in die quantenchemische Modellierung einbeziehen, Bunsen Magazine 6, 204-207 (2023)Speaker: Dr Wilke Dononelli (Universität Bremen) -
15:40
Signal denoising and active learning for multiplexing spectrometer 20m
Inelastic neutron scattering instruments allow detailed studies of the dynamical structure factor, $S(Q, \omega)$, where $Q$ is a scattering vector in reciprocal space and $h\omega = \Delta E$ an energy transfer. One of the work horses of modern neutron scattering is the triple-axis instrument, which typically have a high neutron flux and good energy resolution.Novel multiplexing triple-axis neutron scattering spectrometers yield significant improvements to the common triple-axis concept. Standard triple-axis (TAS) instruments cover only a single $(Q, h\omega)$-position per acquisition time, which leads to isolated trajectories mapped along lines of $\omega$ or $Q$ during experiments. Multiplexing triple-axis instruments extend the triple-axis concept by employing multiple analysers and detectors. This allows for simultaneous measurements of large $S(Q,\omega)$ regions (increased data acquisition rate), while preserving a high neutron flux. Thanks to the recent development of the software package MJOLNIR, we are now able to manipulate and visualize data of the multiplexing spectrometer CAMEA at Paul Scherrer Insitute.
This boost in the data collection rate necessitates a rapid analysis of the scattered neutrons. However, current analysis strategies require experts to manually go through each dataset and mask out spurious background features. Being a notoriously slow process, conventional analysis methods are further based on defining narrow selections around a presumed signal while the rest of the dataset is discarded. In this work, we introduce a regularized model that enables to capture the background noise by separating it from the signal. The key idea is to exploit the rotation invariance property of the background and the sparsity of the signal to enforce a suited decomposition $Y = X+ B$, where $Y$ is the (noisy) observation dataset, $X$ is the captured signal and $B$ the captured background.
Another issue raised with the use of CAMEA is the beam time that must be budgeted carefully among contending experiments. Thus, it is crucial to ensure the optimal use of the time allocated to each experiment through an automated intelligent decision making system that will determine when sufficient data has been collected in an arbitrary measurement setting. To address this issue, we model the dispersion signal as a Gaussian Process that provides uncertainty estimation. Leveraging this confidence level, we can determine a data collection stopping time with respect to a given acceptance threshold. This will help CAMEA users to avoid beamlight overuse when a sufficient amount of data has been collected. We believe that the presented machine learning tools can be adapted to the use of different scattering instrument.Speaker: Dr Daniel Mazzone (Paul Scherrer Institut) -
16:00
Active-learning assisted neutron spectroscopy: challenges and perspectives 20m
Neutron three-axis spectrometers (TAS) provide the opportunity to model lattice dynamics and magnetic interactions by measuring the energy loss of neutron while interacting with material, and applying physical knowledge on the results. In result, the forces forming chemical structures, the origin of magnetic order and the reasons for hybridized excitation modes can be determined qualitatively and quantitatively.
While the technique kept its importance for more than 70 years, it suffers from being expensive and slow, and the very limited availability of beamtime. Therefore, usage of modern techniques is requested to increase efficiency and make better use of given beamtime. Multiplexing is an opportunity for some kinds of experiments, pre-experiment simulations could improve searching strategies (on digital twins as well as theoretical calculations), and the use of advanced computing methods provides alternative experimental strategies.
An active-learning approach based on log-Gaussian process regression was successfully developed for TAS mapping mode measurements (ARIANE, see Nat. Comm. (2023) 14:2246). Here we present our approach and discuss challenges we need to address and perspectives for further developments.
Speaker: Dr Marina Ganeva (Forschungszentrum Jülich GmbH, JCNS)
-
14:30
-
16:20
→
16:50
Coffee Break 30m Bürgerhaus 1 - Bürgerhaus Main ball room
Bürgerhaus 1 - Bürgerhaus Main ball room
Bürgerhaus Garching
Bürgerplatz 9 and Telschowstraße 4, 85748 Garching bei München and MLZ Lichtenbergstr. 1 85747 Garching200Show room on map -
16:50
→
18:40
Session 4 Bürgerhaus 1 - Bürgerhaus Main ball room
Bürgerhaus 1 - Bürgerhaus Main ball room
Bürgerhaus Garching
Bürgerplatz 9 and Telschowstraße 4, 85748 Garching bei München and MLZ Lichtenbergstr. 1 85747 Garching200Show room on map-
16:50
MLExchange: A Machine Learning Platform for On-the-fly Data Analysis at Scientific User Facilities 30m
With the continuous enhancement of experimental capabilities at scientific user facilities, the demand for computational tools that seamlessly guide users through their data lifecycle grows exponentially. These tools play an important role in facilitating the application of machine learning (ML) techniques to accelerate materials discovery. In light of this, MLExchange introduces a collaborative web-based platform to democratize diverse workflows for on-the-fly data visualization, rapid ML-based data analysis, automated experiments, and other applications. Currently, MLExchange offers a selection of web-based graphical user interfaces (GUI) for image segmentation, latent space exploration, data labeling, and classification [1].
In particular, its data labeling pipeline, Label Maker, aims to accelerate the demanding and time-consuming process of labeling scientific data sets through similarity-based querying, clustering, and classification approaches. To achieve this, its architecture connects four independent GUIs: (1) Data Clinic for latent space extraction, (2) MLCoach for data classification, (3) Latent space explorer for dimension reduction, latent space visualization, and clustering, and (4) Label Maker for data visualization and label assignment. Across this pipeline, the web applications make use of an assortment of ML-based techniques, including principal component analysis (PCA) and Uniform Manifold Approximation and Projection (UMAP) for dimension reduction, Density-based spatial clustering (DBSCAN) and Mini Batch K-means for data clustering, and tunable deep learning algorithms for latent space extraction and data classification. Label Maker has shown potential applications for cross-facility learning by using Tiled for data access, which has enabled the visualization of Resonant Soft X-ray Scattering data collected at the National Synchrotron Light Source II. Furthermore, we have successfully demonstrated its effectiveness in enhancing the fine-tuning process of foundational models with human feedback.
Overall, the MLExchange platform offers a collaborative ecosystem to easily deploy ML-based algorithms for scientific data analysis. Among these efforts, MLExchange aims to enhance its capabilities to handle complex workflows, such as mitigating training biases with foundational models and enabling cross-facility model training.
[1] Z. Zhao, T. Chavez, E. A. Holman, G. Hao, A. Green, H. Krishnan, D. McReynolds, R. J. Pandolfi, E. J. Roberts, P. H. Zwart, H. Yanxon, N. Schwarz, S. Sankaranarayanan, S. V. Kalinin, A. Mehta, S. I. Campbell, and A. Hexemer, “MLExchange: A web-based platform enabling exchangeable machine learning workflows for scientific studies,'' in 2022 4th Annual Workshop on Extreme-scale Experiment-in-the-Loop Computing (XLOOP), 2022, pp. 10–15. doi: 10.1109/XLOOP56614.2022.00007
Speaker: Tanny Chavez (Lawrence Berkeley National Laboratory) -
17:20
Learning many-body potential energy landscape for analysis of neutron scattering data 20m
For the discovery of new materials in the field of energy storage, catalysis, and biological processes, molecular dynamics (MD) simulations are an indispensable computational tool. We can achieve highly accurate representations of the potential energy landscape of diverse molecular systems with ab-initio molecular dynamics (AIMD) simulations, but at the cost of high computational time which limits typical applications to 100s of atoms and time scales of ∼100ps. This is where machine learning potentials can be used to capture the underlying physics from first principles, where electrons are treated quantum mechanically, while still reaching long simulation times relatively cheaply.
We introduce a workflow for the analysis of neutron scattering data that trains several deep-learning based many-body potentials and interatomic forces from ab-initio reference calculations. Further, to gauge the accuracy of such potentials with classical MD programs, we use the inelastic neutron scattering (INS) spectra as the performance metric. An INS spectra serves as one of the most stringent tests of theory (such as density functional theory), since the model has to predict not only the correct structure but also the correct vibrational dynamics. We use a genetic algorithm to optimize several hyperparameters used in this workflow. For different molecular samples, we successfully demonstrate that our workflow can replicate the experimental INS spectra measured at SNS.
Speaker: Gaurav Vishwakarma -
17:40
Machine-learning tools for efficient analysis of X-ray scattering data from wood 20m
Wood is a heterogeneous biological material, which has a hierarchical structure extending from the molecular level to the macroscopic scale. X-ray and neutron scattering methods are particularly suited for studying wood, because they cover a large portion of the structural hierarchy and allow characterization of samples under various conditions. Wide-angle X-ray scattering (WAXS) detects the crystalline cellulose microfibrils (2-3 nm in diameter), and small-angle X-ray scattering (SAXS) their cross-sectional size and packing. Wood and other similar plants consist also of different tissue and cell types, which vary in a sample in the microscale and show differences in the scattering data measured at different locations.
State-of-the-art X-ray scattering setups especially at synchrotrons allow gathering large amounts of spatially resolved data. In samples like wood, where the structure varies in the scale of the X-ray beam diameter and above, this would ideally allow scanning-SAXS/WAXS to be measured from different cell and tissue types individually. However, often in reality the scattering contributions of different structures overlap, making them difficult to distinguish from each other. Also, when spatially resolved data from individual tissue types can be collected using a small X-ray beam, the vast amount of data makes it challenging to interpret without further reduction or classification.
Our group is addressing these challenges by implementing machine learning tools for the analysis of X-ray scattering data from woody samples. We have utilized principal component analysis (PCA) and clustering to classify fitting results from a scanning-SAXS/WAXS experiment to find representative results for earlywood and latewood tissues in Norway spruce. We also used PCA and clustering to classify spatially-resolved 2D WAXS patterns, measured by scanning radial cross-sections of wood samples from different species, into different categories according to the tissue type they represent. We also utilized X-ray microtomography to determine the ratio of different cell types in 2D WAXS patterns from bamboo, and used this information to train supervised machine learning models to estimate the same ratio from the scattering patterns directly. The results demonstrate the value of machine learning tools in helping to analyze and interpret large amounts of scattering data measured from heterogeneous biological materials.
Speaker: Dr Paavo Penttilä (Aalto University) -
18:00
Weak-signal extraction enabled by deep-neural-network denoising of diffraction data 20m
Removal or cancellation of noise has wide-spread applications for imaging and acoustics. In every-day-life applications - such as image restoration - denoising may even include generative aspects, which are unfaithful to the ground truth. For scientific use, however, denoising must reproduce the ground truth accurately. Denoising scientific data is further challenged by unknown noise profiles. In fact, such data will often include noise from multiple distinct sources, which significantly reduces the applicability of simulation-based approaches.
We show how scientific data can be denoised via a deep convolutional neural network such that weak signals appear with quantitative accuracy. In particular, we study X-ray diffraction and resonant X-ray scattering data recorded on crystalline materials. We demonstrate that weak signals stemming from charge ordering, insignificant in the noisy data, become visible and accurate in the denoised data. This success is enabled by supervised training of a deep neural network with pairs of measured low- and high-noise data. We additionally show that using artificial noise does not yield such quantitatively accurate results. Our approach thus illustrates a practical strategy for noise filtering that can be applied to challenging acquisition problems.
Speaker: Mr Jens Oppliger (University of Zurich) -
18:20
Machine Learning-Based Reconstruction of Quantitative Compositional Maps in Complex Microstructures: Fusion of Neutron and X-ray Tomography with Raman Analysis 20m
The intricate and unstable nature of corrosion in iron-based materials, such as in archaeological materials, necessitates advanced non-destructive methods for compositional analysis and phase segmentation. The accurate quantitative clustering of these compounds requires a robust analytical framework capable of delineating the various phases present in the thick and irregular corrosion layers. This research presents a multimodal and multi-resolution imaging framework for accurately segmenting and quantifying the phases in corroded iron-based materials, exemplified by Roman nails unearthed from archaeological sites. We employ a workflow that first registers and fuses Neutron (NCT) and X-ray (XCT) tomograms, which are subsequently registered with high-resolution 2D optical microscopy (OM) images. These OM images were acquired on multiple physical cross-sections of these nails and annotated with chemical information obtained by Raman spectroscopy. This 2D-to-3D registration is pivotal in associating non-destructive imaging data with precise phase identification, enabling the accurate labeling of phases within the fused X-ray and neutron tomogram cross-sections (currently only at several 2D cross-sections). This is fulfilled by leveraging a U-Net based deep learning model, adapted for learning the complex relationship between low-resolution and high-resolution labels. This workflow enables discerning and generalizing the identification of these phases, thus achieving a reliable 2D segmentation (creating a composite mapping). Future work will extend these methodologies into three dimensions, aiming for a holistic 3D quantification of corrosion compounds. Such a 3D reconstruction will be reinforced using energy-dependent cross-section curves from energy-resolved time-of-flight measurements and calibration NCT-XCT tomographies from the compressed standard powder pallets of the expected corrosion products in our samples (above 9 expected compounds), ensuring the accuracy of phase differentiation in the three dimensions. The adoption of a V-Net architecture will further enable this extrapolation of the 2D segmented phases into a 3D volume, capturing the full complexity of the corroded samples. After training on several samples, this model should allow us to predict the compositional map of similar samples based on their tomography without the need for further destructive analysis. This method underscores the potential of combining multimodal imaging and deep learning in revolutionizing the analysis of corroded materials, with implications that stretch beyond laboratory applications. The proposed approach will not only enhance our understanding of the material degradation but will also serve as a precursor for our future predictive computational simulations. These simulations will model the behavior of corroded materials under various environmental conditions, aiding the conservation of artifacts and guiding materials engineering practices. Furthermore, the versatility of this framework makes it adaptable to a wide array of material science problems, by offering an approach for a 3D compositional map reconstruction of complex samples, for which to the best of our knowledge there is no existing destructive or non-destructive method.
Speaker: Dr Mahdieh Shakoorioskooie (Applied Materials Group, Laboratory for Neutron Scattering and Imaging at the Paul Scherrer Institute, Forschungsstrasse 111, 5232 Villigen, Switzerland)
-
16:50
-
08:00
→
09:00
-
-
09:00
→
10:30
Session 5 Bürgerhaus 1 - Bürgerhaus Main ball room
Bürgerhaus 1 - Bürgerhaus Main ball room
Bürgerhaus Garching
Bürgerplatz 9 and Telschowstraße 4, 85748 Garching bei München and MLZ Lichtenbergstr. 1 85747 Garching200Show room on map-
09:00
Anomaly detection at an X-ray FEL 30m
Modern light sources produce too many signals for a small operations team to monitor in real time. As a result, recovering from faults can require long downtimes, or even worse subtle performance issues may persist undiscovered. Existing automated methods tend to rely on pre-set limits which either miss subtle problems or produce too many false positives. AI methods can solve both problems, but deep learning techniques typically require extensive labeled training sets, which may not exist for anomaly detection tasks. Here we will show work on unsupervised AI methods developed to find problems at the Linac Coherent Light Source (LCLS). Whereas most unsupervised AI methods are based on distance or density metrics, we will describe a coincidence-based method that identifies faults through simultaneous changes in sub-system and beam behavior. We have applied the method to radio-frequency (RF) stations faults — the most common cause of lost beam at LCLS — and find that the proposed method can be fully automated while identifying 50% more events with 6x fewer false positives than the existing alarm system. I will also show work on a general outlier detection method, including an example of finding a previously unknown beam-scraping event.
Speaker: Daniel Ratner (Stanford, SLAC National Accelerator Laboratory) -
09:30
AI & ML at Diamond – One Year On 20m
In this talk I would like to present an overview of the progress made at Diamond Light Source since the 2023 meeting at the ALS. Since April 2023 we have internally held two workshops in order to come up with a roadmap, which I will discuss, as well as delivering internal training, in collaboration with the Scientific Machine Learning group on our campus.
In addition to this we have identified two key infrastructural areas that we believe require addressing, namely where latency is acceptable and where latency has to be minimised or ‘eliminated’. These two scenarios present distinctly different challenges with regards to the type computing infrastructure required, its proximity to experimental hardware and the software stack that sits on top of the hardware.
With this infrastructure in place we aspire to provide the resiliency and longevity that beamline critical software demands by providing appropriate APIs & hooks, devising guidelines on developer best practice and establishing pathways to software sustainability when deploying algorithms and models at facilities. All of which will also have to provide a flexible working environment for developers allowing for the deployment of pre-existing tools as well as the development of new, novel, algorithms as well.
This endeavour will not be easy; however, I would like to present our ideas to foster both discussion and collaboration in this area as we all look to deploying new algorithms and models more routinely at our respective facilities and, potentially, in a more unified pattern. Preferably this pattern would be somewhat in line with industry standards, to make use of the large amount of resource already being poured into the greater AI & ML space worldwide, albeit tailored to our needs.
Speaker: Dr Tim Snow (Diamond Light Source) -
09:50
Hermes: A Repository for Autonomous Materials Science 20m
In this work we present Hermes, a code repository designed to facilitate the development of autonomous materials science. Many common machine learning algorithms have biases or assumptions that do not account for the physics of many materials science problems. It is therefore often necessary to adapt common machine learning tools into physics-informed algorithms. The idea behind Hermes is to bring together the otherwise disparate efforts in creating these physics-informed machine learning tools into a modular and composable repository. Hermes includes machine learning methods useful for materials science applications such as analyzing measurements and autonomous research campaigns as well as methods for communicating with instruments (or computational methods) and FAIR-ly archiving results. Hermes provides a common syntax and modular tool set to easily construct data analysis pipelines – from controlling the instrument, through all the analysis steps, making predictions, choosing the next experiment to perform, and saving the results along the way. In this way Hermes can be used for: autonomously identifying phase maps with x-ray diffraction, autonomously discovering magnetic ordering temperatures with neutron diffraction, efficiently controlling neutron spin wave echo measurements, and discovering optimal materials with the joint inference of structural and property measurements.
Speaker: Austin McDannald (NIST) -
10:10
Optimizing Beamline Performance: The Bayesian Approach 20m
The BAMline, a beamline for material science research at BESSY II, has been operated by the Bundesanstalt für Materialforschung und -prüfung for over two decades. In the last few years, Bayesian optimization (BO) with Gaussian processes (GP) has been introduced as a transformative method in this setting. This contribution highlights the integration and impact of BO and GP in refining BAMline operations.
We will explore the impact of integrating Bayesian methods, especially when combined with Gaussian processes, on the operational efficiency of the BAMline. Our discussion commences with an overview of the fundamental concepts of active learning and optimization. This is followed by an in-depth analysis of specific case studies, drawing on our direct experience with these innovative methods.
For this our focus is on three key applications:
- Alignment of Optical Elements (DCM and DMM): The implementation of
Bayesian optimization using Gaussian processes has revolutionized the
alignment process for the Double Crystal Monochromator (DCM) and the
Double Multilayer Monochromator (DMM). This approach greatly reduced
manual effort and enhanced the efficiency and effectiveness of the
alignment procedure. - Optimal Spot Selection for Spatial Mapping in XRF Studies: In X-ray
fluorescence (XRF) experiments, determining the most informative
sampling spots is crucial. The introduction of BO, informed by GP,
has significantly improved the selection process, especially in large
or heterogeneous samples. This methodology ensures maximum
information gain, optimizing the balance between comprehensive
mapping and resource management. - Selection of Optimal Energies for XANES Measurements in GEXANES
Geometry: In the specific context of X-ray Absorption Near Edge
Structure (XANES) studies using Grazing Exit (GEXANES) geometry, the
Bayesian approach with Gaussian processes is now utilized to identify
the most effective energy settings. This results in detailed spectral
data acquired in a much shorter time.
We also address challenges and limitations encountered during implementation. Additionally, the versatility of this approach in addressing a range of different research questions will be demonstrated.
Speaker: Martin Radtke - Alignment of Optical Elements (DCM and DMM): The implementation of
-
09:00
-
10:30
→
11:00
Coffee Break 30m Bürgerhaus 1 - Bürgerhaus Main ball room
Bürgerhaus 1 - Bürgerhaus Main ball room
Bürgerhaus Garching
Bürgerplatz 9 and Telschowstraße 4, 85748 Garching bei München and MLZ Lichtenbergstr. 1 85747 Garching200Show room on map -
11:00
→
13:00
Session 6 Bürgerhaus 1 - Bürgerhaus Main ball room
Bürgerhaus 1 - Bürgerhaus Main ball room
Bürgerhaus Garching
Bürgerplatz 9 and Telschowstraße 4, 85748 Garching bei München and MLZ Lichtenbergstr. 1 85747 Garching200Show room on map-
11:00
Autonomous Soft Matter Discovery using X-ray Scattering 30m
Autonomous experimentation (AE) holds enormous promise for accelerating scientific discovery, by leveraging machine-learning to drive experimental loops where the machine selects and conducts experiments. This talk will discuss AE at synchrotron x-ray scattering beamlines. Deep learning is used to classify x-ray detector images, with performance improving when domain-specific data transformations are applied. To close the autonomous loop, we deploy a general-purpose algorithm based on gaussian processes. Several examples of successful autonomous experiments in polymer science will be presented, including the use of AE to explore the non-equilibrium self-assembly of block copolymer thin films into non-native morphologies. Finally, we discuss the intersection of large language models (LLMs) with material discovery.
Speaker: Dr Kevin Yager (Brookhaven National Laboratory) -
11:30
A machine learning-empowered automatic loop centering software tool for macromolecular crystallography beamlines 20m
Synchrotron light source facilities worldwide are evolving into the fourth generation, equipped with diffraction-limited storage rings. These machines generate high quality X-rays with intense brightness, low emittance, ultrafast pulse, and highly coherent beams, offering extreme spatial and temporal resolving power that enables multiscale and ultra-fast characterizations. Consequently, the experimental mode at next-generation beamlines is shifting from static to high-throughput, multimodal, cross-dimensional and dynamic characterizations, greatly facilitating significant scientific outputs. However, regardless of instrumentation advancements, the development of software and algorithms is still lagging behind, becoming the limiting factor to fully unleash the capabilities these facilities have to offer. Cutting-edge light source facilities desire cutting-edge software and algorithms to complement, particularly in key areas such as autonomous beam adjustment and experiment control, intelligent experimental steering and scanning path prediction, data acquisition and orchestration, data analysis and interpretation. Today, we are entering the “fourth paradigm” driven by big data, where artificial intelligence (AI) and machine learning plays a crucial role in advancing scientific discovery (AI for Science). Integrating advanced machine learning techniques into a universal large-scale scientific software system designed for next-generation synchrotron facilities, can ultimately solve the limitations on the software end. This talk first briefly presents our latest effort to develop such large-scale software system (Mamba) designed for China’s first fourth-generation light source; then the talk will introduce our recent work on machine learning-empowered automatic loop centering process using SwinTransformer with YOLO object detection models, which is designed for macromolecular crystallography (MX) beamlines. This subject is influential to reduce the man power and labor manually conducted at MX beamlines if one needs to align the X-ray beam with the sample, especially when there exist multiple samples on a single sample plate. The centering process is divided into two stages: automatic loop detection and intelligent crystal recognition. We are integrating the centering process into the software system Mamba with a graphical user interface (GUI) to streamline the centering process at MX beamlines, forming a dedicated software tool to beamline users with detailed operation tutorials provided. Our most recent developmental progress on the centering procedures and the software tool will both be reported. We hope this talk will facilitate inter-institutional cooperation on the development of machine learning-empowered autonomous experiment control system, particularly on those systems relying on automatic and intelligent machine learning object detection and recognition techniques at photon and neutron (PaN) large scale facilities.
Speaker: Chun Li (Institute of High Energy Physics, Chinese Academy of Sciences) -
11:50
Optimizing Neutron Diffraction Experiments at the Spallation Neutron Source with Temporal Fusion Transformers: A Frontier for AI-Driven Real-Time Decision Making 20m
Neutron time-of-flight (TOF) data at the ORNL Spallation Neutron Source (SNS) contains multidimensional temporal information in diffraction and parameter spaces. The field's current state relies on sequential data reduction and analysis steps, often involving data transfer between different platforms and tools which introduces inefficiencies and hinders the seamless integration of different analysis techniques and workflows.
We are developing an integrated approach to reducing and analyzing single crystal neutron diffraction data recorded in event mode to overcome these challenges. The near-term goal is to enable real-time decision-making for TOPAZ beamline, a high-resolution single crystal TOF Laue diffractometer at SNS. This method harnesses an advanced AI/ML model tapping into the high-performance computing (HPC) resources at the Oak Ridge Leadership Computing Facility (OLCF), which seamlessly synchronizes neutron scattering experiments to enable live data analysis. Our model treats the neutron scattering data at the voxel level to accurately predict the neutron scattering pattern in a 4D temporal-spatial space.
The approach, anchored in a Markovian stochastic process, employs the Temporal Fusion Transformer (TFT) model to optimize experiment time. TFT is an attention-based deep neural network (DNN) model that combines long short-term memory (LSTM) encoding of time series and transformer attention layers, specifically designed to align with the multi-horizon forecasting job, providing greater accuracy and interpretability for predicting neutron scattering patterns at the voxel level in a temporal 4D space. We have developed a hierarchical parallelization approach on the OLCF Frontier supercomputer. Using a subset of the neutron TOF event dataset collected at TOPAZ, our TFT model trained on Frontier could help reduce over-counting by around 30% while achieving similar data quality using less neutron beamtime. The outcomes underscore that the integrated approach using AI/ML and HPC can significantly improve beamline efficiency by processing and analyzing live neutron scattering data in multidimensional scattering and parameter spaces, representing a significant step toward reshaping the landscape of neutron scattering research for real-time experiment steering and automation. Our work advances the Integrated Research Infrastructure (IRI) by bridging the gap between U.S. Department of Energy neutron facilities with the Office of Advanced Scientific Computing Research HPC facilities through the lens of AI/ML. This presentation invites us to join this journey, explore the possibilities, and envision the future of neutron science together.Acknowledgement This work was supported by the Oak Ridge National Laboratory (ORNL) Directed Research and Development Program; the U.S. Department of Energy, Office of Science, Office of Advanced Scientific Computing Research, Applied Mathematics program (FWP: ERKJ387). The neutron scattering data were generated at the Spallation Neutron Source, a DOE Office of Science User Facility operated by the Oak Ridge National Laboratory. The computational results were generated at the Oak Ridge Leadership Computing Facility. ORNL is operated by UT-Battelle, LLC, for the U.S. Department of Energy under Contract DE-AC05-00OR22725.
Speaker: Xiaoping Wang (Neutron Scattering Division, Oak Ridge National Laboratory) -
12:10
Towards Closing the Autonomous Loop at Multiple Facilities: Developing Web-based User Interfaces and Data Infrastructure for Autonomous Experiments and Machine Learning Workflows 20m
Autonomous experiments rely on the seamless integration of control systems, data acquisition, data processing, and optimization frameworks. However, the inherent variability in facility- or beamline-specific infrastructure components poses a challenge for developing more generalizable setups and presents an obstacle for replication studies and cross-facility experiments.
This project focuses on establishing a robust infrastructure for autonomous small- and wide-angle scattering experiments at two different synchrotrons: x-ray scattering beamlines at the Advanced Light Source (ALS, Berkeley) and at PETRA III (DESY, Hamburg), initially the SAXS/WAXS/GISAXS/GIWAXS beamline 7.3.3 at the ALS, and beamline P03, the micro- and nano-focus small- and wide-angle X-ray scattering beamline (MiNaXS) at DESY.
The key components of our infrastructure comprise: pyFAI for azimuthal integration, gpCAM for optimization and uncertainty quantification, Tiled for unified data access, Prefect for workflow orchestration, and a Dash Plotly-based web-interface for initial configuration and monitoring during the experiment. We support reduction workflows for both transmission and grazing-incidence geometry and utilize machine-learning methods to extract the features that facilitate an autonomous loop.Speaker: Wiebke Koepp (Lawrence Berkeley National Lab) -
12:30
Autonomous experiments in neutron scattering 20m
We investigate new concepts for enhancing the data acquisition efficiency of scanning type instruments exploring a multidimensional feature space. We test machine-learning algorithms and probabilistic methods in order to minimize the number of experimental data points, which are required to determine models and model parameters down to precisions defined by the scientists. Data acquisition, interpretation and modeling are part of a closed loop, typical of the concept of Autonomous Experimentation [1]. This approach is opposite to data-driven methods [1], which try to maximize the amount of experimental data and to extract data patterns and physical information with the help of neural networks or similar AI techniques.
Taking classic triple-axis spectrometers (TAS) as representative for scanning type instruments, we develop data acquisition strategies on TAS to explore a scattering function S(Q,) in a four-dimensional feature space, spanned by the momentum space, Q, and the neutron energy transfer axis, ℏω. In the context of experimental design, each invested measuring point reduces the initially available experimental budget, i.e. the total available measuring time, which has to be carefully balanced against the gain of information from this measurement. At present the ubiquitous data acquisition method in TAS experiments is grid scanning (so-called const-Q or const-E-scans). However, we were recently able to show that a non-parametric algorithm like Gaussian Process Regression (GPR) has the potential to steer information more efficiently [2-4]. Based on these recent advances, we anticipate a further gain in efficiency by replacing model-independent strategies (as GPR) with physics informed machine learning. This discipline is very recent and rapidly progressing in various domains of science, where sparse data are combined with machine-learning techniques [5]. Policies need to be worked out in order to locate those points in the feature space which have the highest impact on the differentiation of possible available models and which lead to the fastest convergence in the model parameter space.
References
[1] K.G. Reyes, B. Maruyama, MRS Bulletin 44 (2019), 530-537.
[2] Noack, M.M., Zwart, P.H., Ushizima, D.M., et al. Nat Rev Phys 3, 685-697 (2021).
[3] Teixeira Parente, M., Brandl, G., Franz, C. et al., Nat Commun 14, 2246 (2023). https://doi.org/10.1038/s41467-023-37418-8
[4] Boehm M., Perryman D.E., DeFrancesco A., Scaccia L., Cunsolo A., Weber T., LeGoc Y., Mutti P., in Methods and Applications of Autonomous Experimentation, Chapter 14, edited by Noack M.M and Ushizima P.H., CRC Press 2024, ISBN 978-1-032-31465-5.
[5] G.E. Karniadakis, G.E., Kevrekidis, I.G., Lu, L. et al. Nat Rev Phys 3, 422–440 (2021).Speaker: Mr David Elliott Perryman (Institut Laue-Langevin, 71 Avenue des Martyrs, 38042 Grenoble, France and Institute of Physics, Ecole Polytechnique Fédérale de Lausanne, CH-1015 Lausanne, Switzerland)
-
11:00
-
13:00
→
14:30
Lunch Break 1h 30m Bürgerhaus 1 - Bürgerhaus Main ball room
Bürgerhaus 1 - Bürgerhaus Main ball room
Bürgerhaus Garching
Bürgerplatz 9 and Telschowstraße 4, 85748 Garching bei München and MLZ Lichtenbergstr. 1 85747 Garching200Show room on map -
14:30
→
16:00
Session 7 Bürgerhaus 1 - Bürgerhaus Main ball room
Bürgerhaus 1 - Bürgerhaus Main ball room
Bürgerhaus Garching
Bürgerplatz 9 and Telschowstraße 4, 85748 Garching bei München and MLZ Lichtenbergstr. 1 85747 Garching200Show room on map-
14:30
Autonomous closed-loop experiments through ML-based online analysis in synchrotron beamlines: A case study in x-ray reflectometry 30m
Modern synchrotron beamlines and neutron instruments have undergone significant changes due to technological advances and newly deployed infrastructure. Thus, experiments are becoming more data-intense and data-driven and increasingly relying on online data analysis for efficient use of experimental resources. In this regard, machine-learning (ML) based approaches of specific importance for real-time decision-making based on online data analysis and connected closed loop feedback applications.
Following recent advances in ML-based analysis of x-ray reflectometry we present both, the underlying ML models and concepts as well as the integration into closed loop operation in experiments.
Specifically, we present an approach that incorporates prior knowledge to regularize the training process across broader parameter spaces. This method proves effective in diverse scenarios relying on physics-inspired parameterization of the scattering length density profiles. By integrating prior knowledge, we enhance training dynamics and address the underdetermined (or "ill-posed") nature of the problem. We show that our approach scales well with increasing inverse problem complexity, performing efficiently for an N-layer periodic multilayer model with up to 17 open parameters.Pithan et al., J. Synchrotron Rad. (2023). 30, 1064-1075
Hinderhofer et al., J. Appl. Cryst. (2023). 56, 3-11
Munteanu et al., arXiv.2307.05364Speaker: Linus Pithan (DESY) -
15:00
Cold, warm, warmer, hot! Impact of distance metrics on autonomous experimentation 20m
Artificial intelligence (AI), when interfaced with laboratory automation, can accelerate materials optimization and scientific discovery. For example, it may be used to efficiently map a phase-diagram with intelligent sampling along phase boundaries, or in ‘retrosynthesis’ problems where a material with a target structure is desired but its synthetic route is unknown. These AI-driven laboratories are especially promising in polymer physics, where design parameters (e.g. chemical composition, MW, topology, processing) are vast and where properties and function are intimately tied to design features. However, for AI to operate efficiently in these spaces, they must be ‘encoded’ with domain expertise specific to the problems being tackled. In this talk, we focus on the problem of defining appropriate ‘distance’ metrics to describe differences between functions sampled within a design space. Such functions may be spectroscopic (e.g. UV-Vis absorption, fluorescence, impedance) or scattering profiles (SAXS, SANS) of materials, among others. Traditional ‘distance’ metrics, such as Euclidean and parametric definitions, often fail when important features of the measured functions are subtle and/or when sampling takes place far from the target. We have thus developed a new shape-based similarity metric using Riemannian geometry (Phase-Amplitude Distance) that has been successfully implemented in both retrosynthesis and phase mapping problems. This talk will first discuss the definition of the Phase-Amplitude Distance metric. We then demonstrate its implementation in an autonomous batch retrosynthesis problem using spectroscopic signatures in a model system of metal nanostructures. Finally, we implement the new distance metric in phase-mapping problems involving block-copolymers, polymer blends, and inorganic materials to showcase the broad applicability of the method. Mathematically, these phase maps need to be continuous over the design space and correlations are usually defined by shape-based similarity between profiles. We pose both constraints as a geometric feature of the phase map where continuity is obtained by diffusing the shape-based similarity of SAS profiles via a local geometry defined by the linear operators on the design space.
Speaker: Lilo Pozzo (University of Washington) -
15:20
The NIST Autonomous Formulation Laboratory: Solving Industrial Problems with Multifacility X-Ray and Neutron Scattering and AI 20m
Liquid formulations are ubiquitous, ranging from products such as deicing liquids to food/beverages and biologic drugs. All such products involve precisely tuned composition to enable engineered behaviors, whether that be a drug targeting high-pH tumor areas or a deicing fluid thinning at a specific shear rate so a plane takes off. These engineered responses often involve dozens of interconnected active components ranging from viscosity modifiers to dyes, preservatives, fragrances, etc. This complexity often precludes rational, physics-based optimization of product design in response to changing regulatory/sustainability drivers, for example. This talk will describe the Autonomous Formulation Laboratory, a project based at NIST that is capable of autonomously mixing liquids in arbitrary, n-dimensional composition space and characterizing the resulting formulation using x-ray and neutron scattering in combination with spectroscopy, rheology, and other measurments. This platform is driven by custom, highly flexible open source software that can be used to tackle a variety of different problems, from mapping the bounds of a specific, target phase with high accuracy to maximizing overall phase diagram exploration and everything in between. This talk will describe our development and application of the system to a variety of industrial formulation problems and recent efforts to provide highly robust, flexible data classifiers and data fusion approaches to make the most of multimodal data.
Speaker: Peter Beaucage (National Institute of Standards and Technology) -
15:40
Machine Learning for automatic selection of a nanoparticle analytical model for Small Angle X-Ray Scattering data analysis 20m
Small-angle X-ray scattering (SAXS) is widely used to analyse the shape and size of nanoparticles in solution. A multitude of models describing the SAXS intensity resulting from nanoparticles of various shapes have been developed by the scientific community and are used for data analysis. Choosing the optimal model is a crucial step in data analysis that can be difficult and time-consuming. We propose an algorithm based on machine learning which instantly selects the best model to describe SAXS data. The different algorithms compared are trained and evaluated on a simulated database, consisting of 90,000 scattering spectra from 9 nanoparticle models, that realistically simulates various instrumental configurations.
Deploying a universal solution for automatic nanoparticle model selection is a challenge that raises a number of issues. The diversity of SAXS instruments and their flexibility means that the algorithm must be robust to unseen instrument configurations or to high noise levels. We highlight the poor transferability of classification rules learned on one instrumental configuration to another configuration. We show that training on several instrumental configurations makes it possible to generalise the algorithm, with no degradation in performance compared with configuration-specific training.
Our classification algorithm is then validated on a real data set obtained by performing SAXS experiments on nanoparticles for each of the instrumental configurations, which have been characterised by transmission electron microscopy. Although this data set is very limited, it allows us to estimate the transferability of the classification rules learned from simulated data to real data.
Finally, the use of deep learning automatically leading to poor explainability of results, the issue of user confidence is raised. Thus, there is a need for safeguards to guarantee the detection of outliers data and bad predictions. We propose a method based on deep contrastive learning to implement a prediction confidence indicator and an outlier data detector.Speaker: Mr Nicolas Monge (Xenocs, Université Grenoble Alpes)
-
14:30
-
16:00
→
16:30
Session 8
-
16:00
Machine Learning applied to Material Science 30m
Machine learning (ML) is emerging as a new tool for many different fields which now span, among the others, chemistry, physics and material science [1,2]. The idea is to use ML algorithms as a powerful machinery to identify, starting from big data analysis, subtle correlations between simple elemental quantities and complex material properties and then use these to predict them. This approach can help to screen many material properties directly in-silico avoiding more computational expensive ab-initio calculations and experimental measurements.
However, adapting existing ML architectures to problems in chemistry, physics and material science is not straightforward. Several aspects need to be addressed to improve machine performance which can be summarized into prediction accuracy and generalization. Improving these aspects require to go into the details of the algorithm and analyze the way they learn from a training dataset. This allows to identify which architecture, training algorithm and dataset are relevant for the problem at hand.
In the present talk I will give an overview about several techniques and algorithms spanning from domain adaptation to autoencoders to enhance the performance of machine learning applied to experimental and simulation data analysis.[1] Wei Li, Ryan Jacobs, Dane Morgan Computational Materials Science 150, 454-463 (2018)
[2] G. Pilania, A. Mannodi-Kanakkithodi, B. P. Uberuaga, R. Ramprasad, J. E. Gubernatis & T. Lookman, Scientific Reports volume 6, Article number: 19375 (2016).Speaker: Prof. Alessio Gagliardi (Technical University Munich)
-
16:00
-
16:30
→
16:50
Coffee Break 20m
-
16:50
→
18:50
Posters: Poster Session
-
16:50
UNet-based Segmentation of 3D Volumetric microtomography geological samples 20m
Convolutional Neural Networks (CNNs) have emerged as powerful tools in the field of computer vision, demonstrating remarkable capabilities in tasks such as image classification, object detection, and semantic segmentation. Traditional CNNs are primarily designed for processing two-dimensional 2D images. However, many applications, such X-ray tomography and microtomography, involve volumetric data in the form of 3D images. X-ray microtomography in turn is a non-destructive imaging technique that makes use of X-rays for obtaining high-resolution, three-dimensional visualization of the internal structures of small objects.
This work explores the use of CNNs, specifically focusing on the utilization of 2D-UNet and 3D-UNet architectures since these networks usually rank high among the best performing networks for the segmentation tasks. The objective is to perform semantic segmentation, dissecting the various phases present in 3D microtomography images of geological samples. An objective comparison between the predicted results and ground truth is presented.Speaker: Diego bladimir Haro fernandez (unical) -
17:10
Investigating gold deposition of HiPIMS and dcMS on Polystyrene, Poly-4-vinylpyridine and Polystyrenesulfonicacid 20m
High Power impulse magnetron sputtering (HIPIMS) is a novel industrial relevant deposition technique enabling thin metal layers being coated onto polymers with increased adhesion and density. Compared to conventional direct current magnetron sputtering, no pretreatment is required to achieve these properties. So far there is no report discussing the nucleation and growth process during HiPIMS deposition. In this study, the polymer templates polystyrene (PS), poly-4-vinylpyridin (P4VP) and polystyrene sulfonicacid (PSS) are studied. Even though the polymers are very similar in their structure, it is expected that the distinct different functional moiety influence the kinetics of the initial growth stages of the gold layer. Results of field emission scanning electron microscopy (FESEM), simultaneous in situ grazing-incidence small angle X-ray scattering (GISAXS) and grazing incidence wide angle X-ray scattering (GIWAXS) are presented.
Speaker: Yusuf Bulut -
17:30
CrysFieldExplorer: Rapid Optimization of the Crystal Field Hamiltonian 20m
We present a new approach to the fast optimization of crystal electric field (CEF) parameters to fit experimental data. This approach is implemented in a lightweight Python-based program, CrysFieldExplorer, using Particle-Swarm-Optimization (PSO) and covariance matrix adaptation evolution strategy (CMA-ES). The main novelty of the method is the development of a unique loss function, referred to as the spectrum characteristic loss, which is based on the characteristic polynomial of the Hamiltonian matrix. Furthermore, this optimization technique can be generalized to optimize spin wave excitations by performing optimization on multiple exchange Hamiltonian matrices at multiple Q positions in reciprocal space.
The research at ORNL was supported by the DOE, Office of Science, Office of Advanced Scientific Computing Research (contract No. ERKJ387 to Guannan Zhang), and Office of Basic Energy Sciences, Early Career Research Program (award No. KC0402020 to Huibo Cao under contract No. DE-AC05-00OR22725).
Speaker: Qianli Ma (Oak Ridge National Laboratory) -
17:50
Analysis and Segmentation of Nucleus with U-Net 20m
The nucleus, responsible for a cell's genetic instructions and vital functions such as gene expression and replication, plays a crucial role in understanding cellular mechanisms and discovering problems related to diseases. Even with advanced 3D imaging techniques such as soft X-ray tomography (SXT) providing detailed insights into the structure of cellular components, specifically the nucleus, remains a significant challenge [1-2]. Due to differences in DNA packing, the nucleus exhibits significant variations in its intensity and texture, making it difficult to perform segmentation without manual input. Manual segmentation, requiring specialized expertise, consumes extensive time and resources [1, 3-5]. Automatic and accurate automatic segmentation not only aims to simplify analysis but also holds the potential to unlock a deeper exploration of cellular complexities at statistically significant sample sizes. Addressing these challenges could potentially revolutionize our understanding of cellular functions and pathologies.
Previously, we have shown that by a combination of semi-automatically segmented training datasets and machine learning automatic cytoplasm segmentation of diverse cells is possible [6]. In this study, we further explore deep-learning techniques automatic segmentation of cell nucleus. Utilizing the U-Net [7], and an open-source tool 3D Slicer [8], we use morphological contour interpolation based on a few annotated slices, to create training data and with high efficiency to accurately segment nucleus labeled.
Our work on the automatic segmentation of cell nuclei enables monitoring and analysis of DNA packing associated with aging, disease, environment, or genetics. Using dice coefficient and morphological parameters we show that our pipeline can accurately segment the nucleus of human immune T-cells and murine microglia BV-2 segmentation with potential for automatic segmentation across more divergent cell types.Reference:
[1] M. Harkiolaki, et al., “Cryo-soft X-ray tomography: using soft X-rays to explore the ultrastructure of whole cells,” Emerging Topics in Life Sciences, vol. 2, 2018, p. 81–92, doi: 10.1042/ETLS20170086.
[2] G. Schneider et al., “Three-dimensional cellular ultrastructure resolved by X-ray microscopy,” Nat Methods, vol. 7, 2010, doi: 10.1038/nmeth.1533.
[3] K. Nahas and M. Harkiolaki, “Contour: A semi-automated segmentation and quantitation tool for cryo-soft-X-ray tomography,” Cambridge University Press, 2022, doi: 10.1017/S2633903X22000046.
[4] A. Li et al., “Auto-segmentation and time-dependent systematic analysis of mesoscale cellular structure in β-cells during insulin secretion,” PLOS ONE, vol. 17, 2022, doi: 10.1371/journal.pone.0265567.
[5] D. Mca et al., “3D-surface reconstruction of cellular cryo-soft X-ray microscopy tomograms using semi-supervised deep learning,” 2022, doi: 10.1101/2022.05.16.492055.
[6] A. Erozan et al., “ACSeg: Automated 3D Cytoplasm Segmentation in Soft X-ray tomography” 2022, doi: 10.11588/emclpp.2023.2.94947.
[7] O. Ronneberger et al., “U-Net: Convolutional Networks for Biomedical Image Segmentation,” in Medical Image Computing and Computer-Assisted Intervention, 2015, pp. 234–241. doi: 10.1007/978-3-319-24574-4_28.
[8] Fedorov A., et al., “3D Slicer as an Image Computing Platform for the Quantitative Imaging Network,” in Magnetic Resonance Imaging., 2012, pp. 1323–41. doi: 10.1016/j.mri.2012.05.001.Speaker: Ayse Erozan -
18:10
A Study on Minimizing Measurement Time Based on Active Experimentation for Energydispersive X-Ray Diffraction 20m
Particularly in laboratory XRD measurements, where the intensities in diffraction experiments tend to be low, an adaption of the exposure time to the investigated microstructure is crucial [1]. An adequately defined counting time is crucial if a high number of measuring points is examined in one measuring cycle. Examples of such cases are texture and residual stress measurements. A counting time that is too short can lead to a poor signal-to-background ratio or too dominant signal noise, which makes the subsequent evaluation more difficult or even impossible. It is then necessary to repeat the measurement with an adjusted, usually significantly longer measurement time [2]. Since the first evaluation steps following the measurement are standardized procedures, they provide an interesting approach for intelligent methods directly embedded in the measurement sequence [3,4]. In the present study, different approaches are investigated that analyze the continuously growing data set during an energy dispersive diffraction measurement on an application like that one shown in [5], and terminate the measurements after a sufficient measurement time is accomplished (this being defined based on data quality). Eventually, different selection strategies are proposed that intelligently choose the next point of investigation utilizing key characteristics of prior acquired data. It is shown that such strategies are able to significantly minimize the required measurement time without losing information and, thus, open up the possibility for active experimental design in the process.
References
[1] T. Manns, B. Scholtes: Diffraction residual stress analysis in technical components — Status and prospects, Thin Solid Films (2012), doi:10.1016/j.tsf.2012.03.064[2] B. Breidenstein, S. Heikebrügge, P. Schaumann, C. Dänekas: Influence of the Measurement Parameters on Depth-Resolved Residual Stress Measurements of Deep Rolled Construction Steel using Energy Dispersive X-ray Diffraction, HTM, J. Heat Treatm. Mat., 75 (2020) 6, S. 419-432. DOI: 10.3139/105.110423
[3] K. Dingel, A. Liehr, M. Vogel, S. Degener, D. Meier, T. Niendorf, A. Ehresmann and B. Sick.: AI-Based On The Fly Design of Experiments in Physics and Engineering, IEEE International Conference on Autonomic Computing and Self-Organizing Systems Companion (ACSOS-C) (2021). https://doi.org/10.1109/acsos-c52956.2021.00048
[4] D. Meier, R. Ragunathan, S. Degener, A. Liehr, M. Vollmer, T. Niendorf, B. Sick, Reconstruction of incomplete X-ray diffraction pole figures of oligocrystalline materials using deep learning. In Scientific Reports, 13(1), bl 5410. Springer Nature, 2023, https://www.nature.com/articles/s41598-023-31580-1
[5] A. Liehr, T. Wegener, S. Degener, A. Bolender, N. Möller and T. Niendorf: Experimental Analysis of the Stability of Retained Austenite in a Low-Alloy 42CrSi Steel after Different Quenching and Partitioning Heat Treatments, Adv. Eng. Mater. 2023, DOI: 10.1002/adem.202300380
Speaker: Alexander Liehr (Institute of Materials Engineering (University of Kassel)) -
18:30
A Deep Learning approach for Gamma spot removal in CCD-based neutron imaging 20m
Neutron imaging is a powerful tool for non-destructive investigations in many applications. However, neutron radiography images are frequently degraded due to the interaction between gamma photons or scattered neutrons with the camera sensor. The resulting high intensity gamma spots will interfere the following quantitative analysis and 3D CT reconstruction. There already exist various gamma spots denoising methods using a "Find & Replace" strategy. However, fine parameters tuning is required to achieve a good performance. In this work, we propose a fully convolutional neural network architecture based gamma spot denosier (FCDGSD). Experiments show that our FCDGSD method can achieve similar quality as the start-of-the-art "Find & Replace" method, but provides a roughly 10-fold increase in performance. Moreover, we eliminated the need for human intervention of "Find & Replace" for finding the best hyper-parameters for each experimental setup.
Speaker: Michael Schulz -
18:30
A method to correct angular distortions in Bragg Coherent X-ray Diffraction Imaging 20m
Bragg Coherent Diffraction Imaging (BCDI) is a powerful X-ray imaging technique to reveal 3D strain distribution of crystalline nanoparticles. The method records the 3D diffraction intensity of a nanoparticle slice by slice by incrementally rotating the sample within a very small angular range. The iterative phase retrieval method will then be employed to phase the sampled 3D diffraction intensity and provides the detailed three-dimensional (3D) distribution of strain. Thanks to the coherence produced by the latest $4^{th}$ generation of highly brilliant X-ray beams, BCDI can achieve a very high spatial resolution. However, any angular distortions from nominal rocking angles due to factors like the radiation heating, pressure or the imprecise rotation stage in the data acquisition process can introduce the artifacts in the following phase retrieval, which limits the applicability of BCDI. This prevents us from exploring more in material science, especially for the case of small nanoparticles.
In this study, we introduce a pre-processing algorithm designed to mitigate the impact of unexpected orientations. Inspired by the Extension-Maximize-Compress algorithm commonly employed in single particle x-ray imaging, our approach generates and refines a 3D diffraction intensity volume from measured 2D diffraction patterns. It achieves this by maximizing a likelihood function informed by Poisson statistics. This function includes cross-correlation between photon counts in each measurement and pixels in each slice of the generated volume, facilitating the determination of the relative orientation trajectory. Additionally, we further impose spatial constraint (envelope) on the 3D diffraction volume update, effectively limiting the field of view and enforcing the particle’s maximum physical dimensions.
Our method demonstrates significant resilience to angular distortions, accurately correcting for distortions up to 16.4 times (1640%) of the angular step size $\text{d}θ=0.004^∘$, which is comparable to the fringe spacing in our simulated dataset. The corrected result remarkably improves the quality of subsequent phase retrieval reconstruction, even in presence of Poisson noise.
The validation test underscores the potential of our pre-processing method to recover highly distorted experimental data that would otherwise be unusable. This advantage not only salvages data previously considered lost but also enhances the robustness of BCDI under less-than-ideal conditions. For example, our method can handle the data from the continuous scanning BCDI experiment.
In conclusion, the implications of this work extend to enabling BCDI in more demanding and challenging environments, fully leveraging the intensity of beam from $4^{th}$ generation synchrotrons, pushing the frontiers of material science research.Speaker: Huaiyu Chen (Lund University) -
18:30
A solid-state electrolyte interface layer-amphiphilic polymer/metal composite nanoarray for lithium metal battery 20m
Due to the urgent demand for high-energy-density batteries, lithium (Li) metal batteries (LMBs) have garnered increasing attention. However, the development of LMBs has been hindered by limited cycle life and safety concerns arising from side reactions between lithium metal and the electrolyte, as well as the formation of unstable solid electrolyte interfaces. To address this issue, a nanostructured substrate was fabricated on copper foil by adjusting the ratio of the flexible block copolymer (PS-b-PEO) and LiTFSI. Subsequently, High Power pulse magnetron sputtering (HiPIMS) technology was employed to deposit a layer of gold nanolayer onto PS-b-PEO/LiTFSI, creating an artificial solid electrolyte interface layer of a polymer/metal composite nanoarray.
This experimental setup was conducted using the in-situ scattering device located at the Synchrotron Radiation Center in Hamburg, Germany. On one hand, it facilitated the preparation of the solid electrolyte interface layer in the amphiphilic polymer/metal composite nanoarray. On the other hand, it provided insights into the role of gold nanoparticles in the nanometer-sized particle structural block copolymer surface deposition behavior. The gold nanoparticles within the constructed polymer/metal composite nanoarray's artificial solid electrolyte interface layer effectively reduce the nucleation potential energy, and the polymer substrate helps mitigate the powdering of gold nanoparticles, thereby stabilizing the interface layer and enhancing the stability of the Li||Cu cells.
Speaker: Zhuijun Xu (Technische Universität München) -
18:30
AI-supported preclassification of GISAXS images: mathematical identity detection by autoencoders 20m
Due to the high amount of data during a series of GISAXS measurements, automatic binning is helpful for the evaluation by the researcher. This binning supports the confirmation of existing theories or shows outliers where physics and models need to be refined. Such bins represent unique, relevant parameters of the results, also called identity = “collective aspect of the set of characteristics and properties by which a thing is recognizable or known” [1]. In terms of GISAXS images with 1 mio. Pixels, this identity can ideally be reduced to its few relevant physical parameters such as radius, distance, radius, relative scattering and structure type. However due to the indirect measurement method, the high noise and the randomness of individual electron movements, the resulting image can – currently – only visually be analysed. With our research, use AI methods to overcome the missing determinism of the resulting images and to find unique properties for preprocessing and binning [2].
The overall research question is: How has a multistage AI-supported process been designed and trained to detect and pre-classify GISAXS images?
In our first attempt, we tried Convolutional Neuronal Networks (CNNs) for simulations to generate predictions of nanostructures of experimental GISAXS data [2]. One of the limitations was that the experimental GISAXS data contained noise while the simulations did not. The work identified two options that exist to close the sim-to-real gap: adding noise to the simulations (data augmentation) or trying to remove the noise from the experimental data (denoising). The approach used in [3] was data augmentation. The limitation of this approach is that we need to make sure that the CNN can generalize the noise and simply learn a few specific instances of the possible noise.
Therefore, we decided to use Autoencoders [4]: Autoencoders (AEs) are self-supervised neuronal networks (NNs) divided into an encoder and a decoder network and are used to learn the identity function for the input. A multitude of architecture variations for the AE architecture exist that are used to either prevent the AE from learning the identity function or enhance the ability to capture the important information and create a richer learning representation. One of these variations is the denoising AE (DAE), which removes the noise in an image during the decoding process by learning the noise identity and storing it in its latent space. In our overall processing pipeline, this is a promising first step to understand and model the noise on GISAXS images better. In later steps towards binning, we will use the knowledge about the noise in the latent space to apply this noise to simulation images for further training steps of NNs.
At the conference, we will present the AE architecture and the encouraging results of artificially created, deterministic images in terms of their noise detection, noise classification and identification.
[1] International Standardization Organisation (ISO), “ISO/IEC 20944-1:2013(en): Information technology — Metadata Registries Interoperability and Bindings (MDR-IB) — Part 1: Framework, common vocabulary, and common provisions for conformance,” 2013. [Online]. Available: https://www.iso.org/obp/ui#iso:std:iso-iec:20944:-1:ed-1:v1:en:term:3.21.11.15. [Accessed 13 12 2023].
[2] V. Skwarek, E. Almamedov, S.-J. Wöhnert, S. Dan, A. Rothkirch, M. Schwartzkopf and S. V. Roth, “The role of identities for advanced measurement,” in Gisasx Workshop, Hamburg, 2022.
[3] E. Almamedov, Entwicklung eines Deep Learning Algorithmus zur Bestimmung von morphologischen Identitätsparametern aus GISAXS-Streubildern, Hamburg: Deutsches Elektron Synchrotron & University of Applied sciences Hamburg (HAW), 2022.
[4] S. Dan, E. Almamedov, M. Schwarzkopf, V. Skwarek, T. Chaves, A. Rothkirch and S. V.Roth, “Application Overview for Autoencoder in GISAXS Data Analysis,” in GISAXS Workshop, Hamburg, 2022.Speakers: Eric Schaefer (HAW), Volker Skwarek (HAW) -
18:30
AI/ML-supported evaluation of PGAA data 20m
Prompt Gamma Activation Analysis (PGAA) is a highly sensitive non-destructive method of chemical analysis with neutrons. PGAA is widely used in various scientific fields, such as archaeometry, materials science, and biomonitoring of air pollution. Prompt gamma-ray spectra contain up to thousands of gamma lines, which results in a laborious and time-consuming post-processing by hand. The EvalSpek-ML project will provide a new AI/ML-based approach for the evaluation of convex-combinable spectra. We will present ideas and preliminary results of the PGAA-related work packages. The EvalSpek-ML consortium is a collaboration between Helmholtz-Zentrum Hereon, Technical University of Munich, Helmut Schmidt University Hamburg and AiNT GmbH. Funding is provided by BMBF in the framework of the German action plan “ErUM-Data”.
Speaker: Dr Christian Stieghorst (TUM / FRM II) -
18:30
ALSA: Automatic Laue Sample Aligner 20m
The co-alignment of multiple individual single crystals is a common practice in mass-sensitive techniques like μSR and inelastic neutron scattering, particularly when limited by the ability to grow larger crystals. This alignment process has historically been labour-intensive and often not very precise (e.g. [1]).
The ALSA device aims to revolutionize this procedure by automating the co-alignment process by integrating machine learning and cutting-edge technologies. Utilizing a state-of-the-art X-Ray Laue diffractometer, robotic manipulators, real-time camera recognition, and bespoke neural network software for crystal placing and Laue pattern solving [2], ALSA promises to be a game-changer in the field of sample preparation. It will significantly accelerate the sample preparation process, offering a substantial leap forward in efficiency and precision. To glue small crystals as close to each other as possible, we have developed an online algorithm for irregular polygon stacking; with a series of benchmarking tests proving that it is the most efficient online algorithm available. The whole robotic device uses Bayesian optimization to find the best parameters for achieving the given tasks. In this presentation, we will focus on the design of the device, as well as practical tests on an inelastic neutron spectrometer IN12, where more than 200 irregular single crystals of Na2BaMn(PO4)2 were automatically coaligned with mosaicity below 2 degrees.
[1] Duan, C. Et al. Nature 600, 636–640 (2021). doi:10.1038/s41586-021-04151-5
[2] See abstract "Tackling Laue pattern solving using neural networks"Speaker: Štěpán Venclík (Charles University) -
18:30
Analyzing collective excitations using implicit neural representations 20m
Understanding collective excitations in materials is important for developing the next generation of spintronic devices for information transfer and storage. Excitations are often characterized via the dynamical structure factor, $S(\mathbf{Q}, \omega)$, which can be measured using inelastic neutron or x-ray scattering techniques. Real-time analysis during an experiment is challenging due to the high dimensionality of datasets and the slow nature of theoretical simulations. We present a data-driven tool using 'neural implicit representations' for efficient parameter extraction from inelastic neutron scattering data. By training the tool with linear spin wave theory simulations, we achieve precise Hamiltonian parameter extraction for the square-lattice spin-1 antiferromagnet La$_2$NiO$_4$, highlighting automatic refinement possibilities for ordered magnetic systems [1].
[1] Chitturi, Sathya R., et al. "Capturing dynamical correlations using implicit neural representations." Nature Communications 14.1 (2023): 5852.
Speaker: Mr Sathya Chitturi (SLAC National Accelerator Laboratory) -
18:30
Artificial intelligence methods for synchrotron radiation image and diffraction data analysis 20m
High-energy synchrotron radiation (SR) light sources provide precise and deep insights that have been driving cutting-edge scientific research and innovation in a wide range of scientific fields. High Energy Photon Source (HEPS) is one of the fourth-generation SR light sources and the first one in China. With the advantage of high energy and ultra-low emittance, HEPS will provide more sensitive, finer and faster experimental tools to observe the complex samples and collect multidimensional, real-time and in-situ information at the molecular, atomic, electronic, and spin levels. Along with those advanced techniques, HEPS will generate about 1 petabyte of high-dimensional and complex data per day. Therefore, it is an urgent key scientific and technical challenge to develop artificial intelligence (AI) analysis methods to automatically and efficiently deal with SR data. To address this urgent need and challenge, our researches focus on developing AI analysis methods for SR image and diffraction data. First, regarding image data, we implement a novel localization quantitative analysis method based on deep learning to analyze X-ray nano-computed tomography. We achieve localization quantitative three-dimensional imaging analysis of single-cell HfO2 nanoparticles and demonstrate the notable effect of the nanoparticles in tumor treatment. Our approaches show the potential to explore the localization quantitative three-dimensional distribution information of specific molecules at the nanoscale level. Second, regarding diffraction data, we develop two sets of data-driven and physics-knowledge-driven machine learning (ML) methods to analyze the X-ray diffraction and extract three-dimensional orientation information of nanofibers. The data-driven ML model achieves high accuracy and fast analysis of experimental data and is available to be applied in multi light sources and beamlines. The physics-knowledge-driven ML method enables high-precision, self-supervised, interpretable analysis and lays the foundation for systematic knowledge-driven scientific big data analysis. Overall, our work aims to analyze high-energy SR data quickly and accurately in real-time through advanced AI algorithms, which support AI for SR-based Science strongly.
Speaker: 丽娜 赵 (中国科学院高能物理研究所) -
18:30
Artificial neural networks, operando X-ray powder diffraction CT and functional devices 20m
X-ray chemical tomography methods are non-destructive techniques that provide hyperspectral images of a sample's cross-section. These methods merge spectroscopy or scattering techniques with tomographic data collection, resulting in coloured images containing spatially-resolved physico-chemical information. Each pixel in these reconstructed images corresponds to a complete spectrum or scattering pattern, uncovering information typically lost in bulk measurements. However, these powerful characterization methods generate large datasets. For instance, modern X-ray powder diffraction computed tomography (XRD-CT) experiments at synchrotron facilities produce 100,000s to 1,000,000s of diffraction patterns.
In this presentation, I will show case studies where we have applied synchrotron XRD-CT to examine, under operating conditions, commercially available and industrially relevant samples, like cylindrical Li-ion batteries used in electric vehicles. Additionally, I will discuss cutting-edge deep learning developments we have been recently making, including ultra-fast data analysis, self-supervised data denoising and tomographic image reconstruction.
Speaker: Antonis Vamvakeros (Imperial College London / Finden ltd) -
18:30
Assisting users on model selection in small angle neutron scattering experiments 20m
Nowadays software for Small Angle Scattering (SAS) data fitting have a large selection of analytical and numerical models to describe the form factor of the scattering objects. It may become overwhelming to choose an adequate model for the data, especially for new users of SNS instruments. In this work, we train a convolutional neural network (CNN) to predict the form factor model on a dataset comprising of 300.000 SANS 2D images obtained by means of virtual experiments. The CNN hast a 94% of accuracy in the classification task, and a 99.9% top 3 accuracy, i.e. that the probability of the model not being amongst the 3 recommended models (out of the 47 in the database) is 0.1%. We explain the dataset creation,and the training procedure. Once trained, these algorithms can run on the fly, while performing measurements on an instrument. We also show the use of explainable machine learning algorithms, in particular SHAP and Grad-CAM, to interpret how the CNN is making decisions.
Speaker: Jose Robledo (JCNS-2 Forschungszentrum Jülich) -
18:30
Automatized data analysis for surface XRD 20m
Understanding structure-property relationships in structural materials can only advance with state-of-the-art characterization. Probing the structure by x-rays has only recently become feasible, mostly by advances in nano-focusing. By scanning techniques, diffraction data of many different grains can be collected. My project aims at dealing with the data obtained from such experiments, in particular automated diffraction spot analysis and data reduction of 2D detector images using machine learning methods.
Speaker: Radik Batraev (Nanolab (Photon Science, DESY)) -
18:30
Biopolymer-Templated Deposition of Hierarchical 3D-Structured Graphene Oxide/Gold Nanoparticle Hybrids for Surface-Enhanced Raman Scattering 20m
Cellulose, a well-known natural biopolymer, possesses numerous advantages such as cost-effectiveness, renewability, ease of processing, and biodegradability [1]. Due to these inherent merits, cellulose has emerged as a promising bio-based substrate capable of synergistically combining with conductive materials (e.g., metals or carbon-based materials) for diverse applications including sensors, smart windows, and bioelectronics [2]. Typically, surface-enhanced Raman scattering (SERS), an advantageous analytical technique, allows for the rapid detection and structural analysis of biological and chemical compounds through their spectral patterns in nanotechnology [3]. Crucial for SERS is fabricating the substrates with strong and reproducible enhancements of the Raman signal over large areas and with a low fabrication cost. Herein, we present a straightforward approach utilizing the layer-by-layer spray coating method to fabricate (CNF) films loaded with gold nanoparticles (AuNPs) and graphene oxide (GO) to serve as SERS substrates. To investigate the fundamental mechanisms of enhanced SERS performance, grazing incidence small-angle X-ray scattering (GISAXS) technique combined with the machine learning random forest method is employed to identify different nanostructures for predicting vibrational frequencies and Raman intensities. Therefore, our approach provides a reference for facile and scalable production of universally adaptable SERS substrates with exceptional sensitivity.
Speaker: Yingjian Guo (Deutsches Elektronen-Synchrotron DESY) -
18:30
Characterization of Mesoporous Zinc Titanate Hybrid Films Using Grazing Incidence Small-angle X-ray Scattering 20m
Mesoporous films consisting of zinc titanate have high potential applications in photocatalysis, solar cells, and sensors due to tailoring their semiconductive properties. This study investigates the morphologies of mesoporous zinc titanate films obtained by changing the ratio of two inorganic precursors after calcining hybrid films consisting of organic-inorganic materials. The amphiphilic diblock copolymer poly(styrene)-b-poly(ethylene oxide) PS-b-PEO self-assembles into core-shell micelles in a mixture of N, N-dimethylformamide/ hydrochloric acid playing the role of a structure-directing template. The inorganic precursors, zinc acetate dihydrate and titanium isopropoxide are loaded in the micellar shell due to hydrogen bonds between PEO and precursors. We use slot-die and spin-coating methods to prepare hybrid films and investigate the influence of the different deposition methods on the film morphologies. Moreover, we investigate how mesoporous structures and crystal phases depend on calcination temperature, concentration, and the ratio of two precursors. The morphologies of the hybrid films were characterized using grazing incidence small-angle X-ray scattering (GISAXS) and scanning electron microscopy (SEM) and the obtained data will be used as training data for machine learning approaches.
Speaker: Yanan Li -
18:30
Conformation of star-like molecular brushes with amphiphilic diblock copolymer side arms 20m
The core-shell micelles formed by weakly amphiphilic diblock copolymers from poly(2-oxazoline)s (POx) have been shown to be efficient drug carriers [1]. The water solubility of POx is controlled by the nature of the side groups. In the present work, we investigate POx-based molecular brushes, in which diblock copolymers from hydrophilic poly(2-methyl-2-oxazoline) (PMeOx) and weakly hydrophobic poly(2-n-butyl-2-oxazoline) (PnBuOx) are grafted onto backbones which are star polymers from poly(methyl methacrylate) having functionalities ranging from 2 to 5. The size and shape of the star brushes were investigated in dilute aqueous solution using synchrotron small-angle X-ray scattering (SAXS). We find that all SAXS data can be modeled by the form factor of homogeneous ellipsoids, potentially due to the low X-ray contrast between PMeOx and PnBuOx. Their sizes do not depend on the functionality of the stars, hence, it is not a key factor for the star brush conformation. Also, large aggregates (> 100 nm) are observed for all star brushes even in dilute solutions.
[1] A. Schulz, C. M. Papadakis, R. Jordan et al., ACS Nano 2014, 8, 2686.
Speaker: Wenqi Xu (Technical University of Munich, TUM School of Natural Sciences, Soft Matter Physics Group) -
18:30
Deciphering SASE X-ray Pulse Characteristics with β-Variational Autoencoder Networks 20m
Free electron lasers (FEL) play an important role across diverse scientific disciplines. Many experiments can benefit from a non-destructive online photon diagnostic of provided X-ray pulses. One method to obtain information about the pulse profile involves analyzing not the X-ray photons directly, but rather the energy distribution of the electrons downstream of a Self-Amplified Spontaneous Emission (SASE) undulator.
In recent times, neural networks have gained widespread recognition as potent analytical tools spanning various scientific domains. Among these, β Variational Autoencoder (β-VAE) networks stand out for their ability to discern key parameters within unlabeled datasets, even when these parameters are unknown beforehand.
This study showcases the application of β-VAEs in characterizing SASE X-ray pulses generated by the free electron laser FLASH in Hamburg. Leveraging data from a Transverse Deflecting Structure (TDS), we demonstrate the β-VAE's capacity to identify the SASE strength, a critical parameter, within real-world data from FLASH. This discovery holds promise in improving the accuracy of lasing off references and therefore enhancing the reconstruction of XUV power profiles.Speaker: Gesa Goetzke (Gesa) -
18:30
Deep learning based approach for speeding up the extraction of morphological parameters in GISAXS data 20m
In situ grazing incidence small angle X-ray scattering (GISAXS) is a powerful tool for accessing nanoscale structure formation in real time with high time resolution and high statistical relevance. However, the analysis is clearly time consuming and challenging task, necessitating the need for strategies to speed up the process. In this context we introduce a two-step method that incorporates a pre-processing of GISAXS simulations, which are employed to train a neural network (NN). The NN is subsequently utilized to predict the average cluster radius and distance of the model system gold on silica. There are multiple aspects of the method that require detailed characterization. Here, we focus on the effects of using intensity thresholds in the pre-processing step and on the relationship between the network architecture and the distribution of results. As part of ongoing research, we are investigating different configurations and examining their direct impact on the predictive capabilities of the NN. This iterative refinement process aims not only to improve the effectiveness of the approach for the specific system, but also to lay the foundation for its applicability to broader material systems in the field of GISAXS data analysis.
Speaker: Shachar Dan (DESY) -
18:30
Deep Live Phase Retrieval for Ptychography 20m
Live reconstruction algorithms for ptychographic phase retrieval can enable immediate visual feedback during scanning, allowing for readjustments of the experiment, as well as paving the way for adaptive scanning techniques. We have shown in previous works that live variants of projection-based iterative algorithms, such as the Difference Map, can be naturally derived and may achieve higher quality than their classic non-live counterparts. In this work, we will extend these developments by combining them with another previous work on deep learning augmented projection algorithms. We will specifically adapt modern DNN architectures to this live setting to realize fast, high-quality reconstructions. We will investigate the possible increases in convergence speed, robustness to noise, and ability to perform with low-density scans (few measurements).
Speaker: Simon Welker (Universität Hamburg (Signal Processing) / Center for Free-Electron Laser Science (Coherent Imaging Division)) -
18:30
Developments in Event Mode Data Analysis 20m
Event mode data acquisition in neutron and x-ray scattering experiments has already been demonstrated at multiple labs. The main advantage of this technique is that the data reduction is completely flexible after the experiment, so the re-binning of histograms can be tuned to the experimental data. Compared to accumulating histograms in hardware, event mode data acquisition carries orders of magnitude increases in storage and processing requirements, but since storage and processing have become relatively cheap the advantages tend to outweigh the disadvantages. The routine method of analysis is historically based around least-squares fitting. Since any histogram process discards some information along one axis or plane, because of the integration within each bin, by re-sampling with variable histogram bin widths (or equivalently tuning of the Kernel Density Estimation bandwidth) one can maximise the extracted Fisher information - in other words, reduce the uncertainty on the extracted parameters of interest. In this work-in-progress study, we describe a new project to abandon entirely the histogram step and analyse the event data directly. We give examples that are based on weighted Maximum Likelihood Estimation, Bayesian Inference, and Maximum A Posteriori approaches. We will demonstrate the technical challenges and share our identified pitfalls, along with the successes and most promising steps for future development. Such an approach may be particularly attractive for kinetic experiments of sub-second processes, even perhaps single pulse measurements, where the repeatability of the study is almost impossible, for example destructive processes with weak signals and/or large experimental backgrounds.
Speaker: Phillip Bentley (European Spallation Source ESS) -
18:30
EFFECT OF PEROVSKITE NANOCRYSTAL NUCLEATION SEEDS ON MICROSTRUCTURE AND CRYSTALLIZATION PATHWAYS IN ORGANIC-INORGANIC HALIDE PEROVSKITE THIN FILMS 20m
Organic-inorganic halide perovskites have gained a huge interest in the scientific community owing to their favorable optoelectronic properties combined with their ease of production and abundance of raw materials. [1] In many cases, polycrystalline thin films are used for which thin film crystallinity and morphology are key factors affecting the perovskite’s properties. Various methods have been utilized to improving the mentioned factors [1] from which we present a novel approach employing external perovskite nanocrystals as seeds for printed thin films and present their influence on crystallization kinetics and microstructure based on in-situ grazing incidence wide angle X-ray scattering (GIWAXS) measurements conducted at beamline P03, PETRA III synchrotron DESY, Hamburg [2].
[1] C. Lin et al, Adv. Funct. Mater. 29., 1902582 (2019).
[2] A. Buffet et al, Journal of Synchrotron radiation, 19.4, 647-653 (2012)Speaker: Mr Altantulga Buyan-Arivjikh (Technical University of Munich, TUM School of Natural Sciences, Department of Physics, Chair for Functional Materials) -
18:30
Energy-Dispersive Detection of X-rays with CMOS Cameras: A Machine Learning Approach 20m
X-ray fluorescence spectroscopy and scattering techniques are pivotal in numerous scientific fields, enabling detailed examination of structures ranging from biological tissues to advanced materials. Traditionally, Charge-Coupled Devices (CCDs) and Complementary Metal-Oxide-Semiconductor (CMOS) sensors have been employed extensively in detecting soft and tender X-rays in various X-ray experiments. These devices have been instrumental due to their high sensitivity and resolution. However, usually the X-ray intensity on the detector is integrated to increase signal to noise ratio. This mode of operation, inevitably, leads to the loss of energy information of the detected photons.
An intriguing possibility arises if individual photons can be distinguished on the detector image. This capability enables energy-dispersive operation of standard CMOS or CCDs through software-based evaluation [1], allowing for “noise-free” detection, effective suppression of background signals or even paving the way for novel methodologies like scanning-free grazing emission X-ray fluorescence. Classical algorithms currently used for this purpose primarily focus on summing up pixel intensities. However, these methods are hindered by their susceptibility to spatial overlap, also known as pile-up, which can distort the data and lead to inaccuracies in interpretation.
An alternative to these conventional methods is the use of intensity pattern fitting. While this approach can be more precise, it is considerably slower, problematic especially when dealing with large datasets typically encountered in energy-dispersive operation of CCDs and CMOS detectors.
In light of these challenges, this poster introduces a novel deep learning approach for accurately determining the position and energy of photons detected by CMOS cameras. We employ convolutional and fully-connected neural networks, trained exclusively on simulated data. The models excel in addressing pile-up issues and enable swift image evaluation.[1] Baumann et al. J. Anal. At. Spectrom., 2018, 33, 2043-2052
Speaker: Dr Jonas Baumann (Technical University of Berlin, Institute of Optics and Atomic Physics) -
18:30
Enhanced air stability of Tin‑Based Perovskite Solar Cells with Quercetin 20m
As the most essential alternative materials for eco-friendly perovskite solar cells (PSCs), Tin-based perovskites have achieved an efficiency of 14.81%, which is far less than 25.7% of lead-based devices. The main reason is that it is easy to oxidize to Sn$^{4+}$ in the presence of oxygen and water due to the low stability of the Sn$^{2+}$ state. The oxidation of Sn$^{2+}$ will form the Sn (Ⅳ) vacancies in the perovskite structure, leading to p-type self-doping and introducing additional defect states. These defects can enhance the device's leakage current and carrier recombination, limit the increase of open circuit voltage, and thus reduce the battery's efficiency. Here, we plan to introduce Quercetin, a phenolic derivative with antioxidation properties extracted from plants, as the additive to reduce the existence of Sn$^{4+}$ and prevent the FASnI$_{3}$ film from degradation. We also use grazing-incidence wide-angle X-ray scattering (GIWAXS) to gain insight into the detailed steps of the growth and degradation progress of the active layer. Thereby, GIWAXS offers a way to gain information about the time evolution during the crucial steps of interface formation. The ultimate goal of our work is to design efficient and stable tin-based PSCs to develop a systematic and reproducible strategy for air stability and high-efficiency PSCs.
Speaker: Xiaojing Ci (Technische Universität München) -
18:30
Establishing Multi-resolution, Multi-modal Data Fusion of Synchrotron Imaging Modalities at Diamond Light Source 20m
In the medical imaging field, DLNs have allowed for many recent advances in imaging processing such as super-resolution and segmentation tasks. Similar applications have been studied in the fields of digital rocks, and Li-ion battery research with super-resolution deep learning models being successfully deployed to enhance the resolution of rock X-ray CT (XCT) images and microscopy images of Li-ion electrodes. The Diamond Light Source produces a significant quantity of imaging, scattering, and spectroscopic data across all 33 beamlines, with expectations of 100s of petabytes (PBs) of data generated when Diamond-II comes online. DLNs have found increasing applicability at the synchrotron in helping to process and analyse this data, across the range of modalities (imaging, scattering, spectroscopy) and length scales (e.g. using phase information from diffraction data to assist image segmentation, or overcoming the physical limitation of certain detectors).
Super-resolution tasks help to overcome limitations of the beamline detectors, such as the field of view (FOV) with super-resolution deep learning models being used to enhance the resolution of larger FOVs that are otherwise not possible due to limitations of experimental equipment. Obtaining a super-resolution dataset at a larger FOV can then allow for cross-correlation of datasets between beamlines, with a pathway for multimodal data fusion through the combination of different modes across different beamlines. Such an example includes combining higher-resolution XCT data with X-ray diffraction CT (XRD-CT). This multimodal dataset could be used for high-resolution segmentation tasks that require no manual annotation, by using the phase information acquired from XRD-CT to train the segmentation DLN. (Mock-up example shown in attached Figure)
In our previous work, we successfully developed and used a super-resolution generative adversarial network (SRGAN) to enhance the resolution of artificially downsampled XCT zeolite datasets acquired on the Dual Imaging and Diffraction beamline (DIAD/K11). Lab-based XCT datasets of porous media have also shown promise in enhancing the resolution of XCT using our developed SRGAN methods, utilising a fully paired, spatially correlated, high and low-resolution dataset that has been experimentally obtained. These datasets demonstrate the feasibility of applying our super-resolution techniques to synchrotron-based XCT datasets as part of our in-development cross-beamline XCT fusion pipeline for automatic XCT segmentation using X-ray diffraction (XRD) as a ground truth.
Speaker: Calum Green (Imperial College London) -
18:30
Exploring the Efficacy of Machine Learning in Interatomic Potentials and Neutron Scattering Spectra Predictions 20m
Machine learning-based atomic potentials have become instrumental in forecasting the structure and dynamics of diverse materials. These potentials, claiming "ab initio accuracy with the efficiency of classical force field," raise questions about their true generalizability across a broad spectrum of materials. This generalizability, a key factor in extrapolating learned information to new systems, minimizes the need for
extensive training data for each material, enhancing efficiency and applicability. To assess these claims, we decided to rigorously test various machine learning-based atomic potentials, calculating structure and lattice dynamics properties for a dozen intriguing systems and comparing results to our own neutron spectra data. In the first part of the talk, we will discuss our findings. In the second part of the presentation, we explore an alternative approach using the Atomistic Line Graph Neural Network (ALIGNN) method to directly predict inelastic neutron spectra without phonon calculations. By training on extensive datasets from the JARVIS database, ALIGNN learns intricate patterns and correlations, providing a swift and efficient alternative to traditional simulation methods to predict INS spectra for a large number of systems.Speaker: Taner YILDIRIM (NIST Center for Neutron Research, National Institute of Standards and Technology (NIST)) -
18:30
Exploring the limits of the random forest algorithm for the classification of X-ray absorption spectra 20m
X-ray absorption spectroscopy gives access to a wealth of information regarding the local structure and electronic properties of materials. However, data analysis is significantly more time-consuming than the acquisition and initial data reduction. Decoding the information relies on comparison with similar compounds for which the spectrum–property mapping is already established, a task that is very often performed by visual inspection.
Machine learning (ML) is revolutionizing many fields with its ability to extract and learn patterns in big data without having to provide additional prior information other than the data itself. ML models give access to instantaneous predictions of properties and observables, which makes them particularly attractive for performing autonomous experimental acquisitions or real-time analysis of the measured data.
Current ML applications for X-ray spectroscopy mainly use neural networks (NN), which require extensive computational datasets as training data.$^{1,2}$ These are very time-consuming to build and are often linked to large-scale computing facilities access. Alternatively, one can use tailor-made ML models that are less data-hungry and can be trained significantly faster. One such ML algorithm is the random forest, which has already shown promising applications for analyzing visible and infrared spectra.$^3$
In the present contribution, we will present the application of the random forest algorithm to identify the coordination environment of iron in a given compound from the corresponding K-edge X-ray absorption spectrum. As we train the model using theoretical data, but we use it to infer properties on measured spectra, we analyze the different sources of errors that limit the quality of the prediction, such as spectral shift, normalization, and noise level. In addition, we explore the use of SMOTE to tackle the class imbalance, a common issue in such datasets as most materials in nature tend to adopt a small set of specific coordination environments.
[1] Timoshenko, J.; Anspoks, A.; Cintins, A.; Kuzmin, A.; Purans, J.; Frenkel, A. I. Phys. Rev. Lett. 2018, 120 (22), 225502. https://doi.org/10.1103/PhysRevLett.120.225502.
[2] Rankine, C. D.; Madkhali, M. M. M.; Penfold, T. J. J. Phys. Chem. A 2020, 124 (21), 4263–4270. https://doi.org/10.1021/acs.jpca.0c03723.
[3] Lee, S.; Choi, H.; Cha, K.; Chung, H. Microchem. J. 2013, 110, 739–748. https://doi.org/10.1016/j.microc.2013.08.007.Speaker: Marius Retegan (ESRF) -
18:30
Gaussian processes regression for preliminary data evaluation at DNS 20m
DNS is a polarised diffuse neutron scattering instrument with a time-of-flight inelastic scattering option at MLZ. DNS is particularly useful and powerful for unraveling momentum-, energy-, and neutron-polarisation resolved magnetic correlations in complex magnetic materials and exotic quantum magnets.
The crucial part of DNS data processing workflow is data reduction, e.g. correction of the collected data by various experimental artifacts caused by the instrument itself or its environment. This step is necessary for a proper assessment of the measured data and steering the experiment. However, calibration data required to perform the data reduction are not always available at the very beginning of the beam time - the most critical time to define the right setting for a successful neutron experiment.
In our work, we develop a procedure that enables DNS instrument scientists and users to perform a preliminary evaluation of the collected data. This procedure employs a Gaussian processes regression approach to preform a simulation of the calibration data using the set of legacy calibration data collected over the various reactor operation cycles. The simulated data can then be used by the users of the ''DNS Reduction'' interface in their preliminary examination of the sample data and, if necessary, adjust some parameters of their experiment during the measurement. Besides being able to use simulated predictions of calibration corrections, the users are also provided with a quantitative estimate of the corresponding uncertainty.
Speaker: Oleksandr Koshchii (JCNS at MLZ, Forschungszentrum Jülich GmbH) -
18:30
Generative models for Inverse Problems: The phase retrieval problem from a single distance intensity measurement 20m
The phase retrieval problem is a non-linear, ill-posed inverse problem. It is also an important step in X-ray imaging, a precursor to the tomographic reconstruction stage. Experiments involving micro and nanometer-sized objects usually have weak absorption and contrast. This is usually the case in most experiments taking place at high-energy big Synchrotron centres like DESY. Hence, retrieving the phase information is crucial for the quality of the tomographic reconstruction. This problem exists also in other fields like astronomy, optics, and electron microscopy. Our research deals with single distance, near or holographic region intensity images, which, in the mathematical sense, are the squared modulus of a complex object that was propagated forward to a certain distance using the Fresnel operator. In this talk, we want to challenge the listeners that generative models can be powerful tools in inverse problems, especially those with clearly defined forward models. We would further show that it plays an important role in uncertainty quantification.
Speaker: Dawit Hailu (Helmholtz-Zentrum hereon) -
18:30
Grazing-Incidence X-ray Scattering Data for Machine Learning of Neural Networks 20m
Relationships between the structure and function are at the heart of material science based on functional films, which makes the knowledge of how film morphology influences its function essential. Key objectives are understanding the formation during synthesis and deposition processes as well as the degradation and the deformation during operation in devices and external stimuli. Achieving a comprehensive and statistically relevant knowledge of the film's characteristics often requires the use of indispensable tools like grazing-incidence X-ray small-angle and wide-angle scattering (GISAXS/GIWAXS). These methods enable the exploration of the film's characteristic morphology in reciprocal space, providing precious insights in a non-destructive way with high time resolution at synchrotrons. However, a challenge arises due to the loss of phase information during measurements, which inhibits a direct transformation from reciprocal space to real space via inverse Fourier transform. In addressing this obstacle, neural networks emerge as promising solutions. The data derived from GISAXS and GIWAXS conducted, e.g., in-situ during formation of functional films, alongside the evaluation utilizing established models, can serve as valuable training sets for the development and refinement of neural networks. For this, two different in-situ data sets obtained from slightly different samples measured at else exactly same conditions regarding the instrumental setup are compared. The first data set is used for training of the neural network and to identify typical features and artefacts in X-ray scattering, as well as distinguishing Poisson noise from the data of interest in complementation to simulated data and results obtained from established models. The trained network is then used to analyze the second data set accordingly and rated with respect to its uncertainty. This strategy holds the potential to enhance our understanding of the structure-function relationships within functional films by enabling the interpretation of reciprocal space data in terms of real-space morphology by neural networks.
Speaker: Julian Heger (Technical University of Munich, TUM School of Natural Sciences, Department of Physics, Chair for Functional Materials) -
18:30
Hamiltonian-driven modeling using image-recognition-based neural networks in rare-earth spin systems 20m
Neutron scattering technique is an ideal tool to observe spin orders and dynamics, primarily governed by the exchange Hamiltonian. Modeling neutron scattering data involves optimizing the Hamiltonian. Traditionally, forward calculations with a proposed Hamiltonian are used to model inelastic or diffuse neutron scattering data, which is achieved by directly fitting the energy and intensity information of selected one-dimensional (1D) slice-cut data from the measured 4D data space. A standard chi square loss function is usually used. However, the efficient and accuracy of this method is highly dependent on the specific magnetic system. Here, we demonstrate the capability of convolutional based encoding-decoding neural networks to model 3D diffuse scattering and 4D inelastic neutron scattering data and directly extract the exchange parameters of Hamiltonian that best fit the experimental data. Specifically, a variational auto-encoder is built to project an inelastic neutron scattering data to a low-dimensional latent space, and a fully connected neural network is coupled with the autoencoder to define a functional map between the parameters of the Hamiltonian and the latent variables of the autoencoder. The antoencoder and the fully connected neural networks are then jointly trained on the synthetic data. After training, the ML model can directly map an inelastic measurement to the corresponding parameters of the Hamiltonian. We selected the rare-earth spin systems for this demonstration due to its complex magnetic interaction matrices.
The research at ORNL was supported by the DOE, Office of Science, Office of Advanced Scientific Computing Research (contract No. ERKJ387 to Guannan Zhang), and Office of Basic Energy Sciences, Early Career Research Program (award No. KC0402020 to Huibo Cao under contract No. DE-AC05-00OR22725).
Speaker: Dr Huibo Cao (Oak Ridge National Laboratory) -
18:30
Hierarchical Bayesian approach for adaptive integration of Bragg peaks in time-of-flight neutron scattering data 20m
The Spallation Neutron Source (SNS) at Oak Ridge National Laboratory (ORNL) operates in the event mode. Time-of-flight (TOF) information about each detected neutron is collected separately and saved as a descriptive entry in a database enabling unprecedented accuracy of the collected experimental data. Nevertheless, the common data processing pipeline still involves the binning of data to perform analysis and feature extraction. For weak reflections, improper binning leads to sparse histograms with low signal-to-noise ratios, rendering them uninformative. In this study, we propose the Bayesian ML approach for the identification of Bragg peaks in TOF diffraction data. The method is capable of adaptively handling the varying sampling rates found in different regions of the reciprocal space. Unlike histogram fitting methods, our approach focuses on estimating the true neutron flux function. We accomplish this by employing a profile fitting algorithm based on the event-level likelihood, along with a multiresolution histogram-level prior. By using this approach, we ensure that there is no loss of information due to data reduction in strong reflections and that the search space is appropriately restricted for weak reflections. To demonstrate the effectiveness of our proposed model, we apply it to real experimental data collected at the TOPAZ single crystal diffractometer at SNS.
Speakers: Guannan Zhang (Oak Ridge National Laboratory), Viktor Reshniak (Oak Ridge National Laboratory), Xiaoping Wang (Oak Ridge National Laboratory) -
18:30
In situ GISAXS investigation of sputtering IZO thin film for optoelectronic applications 20m
Transparent conducting oxide (TCO) thin films have been studied intensively for optoelectronic devices, such as photodetectors, photovoltaics and light emitting diodes (LEDs). Among the several TCO thin films, zinc doped indium oxide (IZO) has received much attention as interface layer in optoelectronic devices due to its excellent electrical conductivity, optical transmittance, high thermal/chemical stability, low cost and low deposition temperature. Here, ITO glass and spin coated ZnO on ITO glass are used as the templates for IZO thin film deposition via DC magnetron sputtering technique. The growth dynamics of IZO film on these two templates are respectively investigated via grazing small angle x-ray scattering (GISAXS) characterization, and the morphology and optoelectrical properties of final films are further investigated.
Speaker: Huaying Zhong -
18:30
In situ GISAXS investigation of ternary hybrid diblock copolymer thin films containing two types of magnetic nanoparticles 20m
The fabrication of hybrid thin films can be realized utilizing diblock copolymers (DBCs) that form periodic, ordered nanostructures and inorganic nanoparticles (NPs). While binary hybrid films have been the focus of much research, ternary hybrid films containing two types of NPs expand the possible functionalities of such films. In this work, polystyrene-block-poly(methyl methacrylate) (PS-b-PMMA) thin films containing iron oxide and nickel oxide nanoparticles are fabricated in a one-step slot die-coating process. The morphology evolution of the hybrid thin films is tracked in situ utilizing grazing-incidence small-angle X-ray scattering (GISAXS). Film formation can be characterized by three stages, clearly observed in the scattering data. The first stage is the wet film, where, after deposition, the scattering from the deposited solution dominates. In the second stage, a rapid coalescence and microphase separation are observed which is unperturbed by the presence of the NPs. Finally, the third stage is attributed to the stable dry film where no further changes in scattering intensity or film morphology occur. The magnetic properties of the hybrid thin films are investigated utilizing a superconducting quantum interference device. With increasing nickel oxide NP content, while maintaining the iron oxide NP content, the saturation magnetization, remanence, and coercivity of the hybrid films are improved. These hybrid films demonstrate the potential for ternary composites to exert a greater degree of control on the resulting magnetic properties.
Speaker: Christopher Everettt -
18:30
In Situ GIWAXS of Slot-Die Coated Perovskite Quantum Dot Thin-Films 20m
Perovskite Quantum Dot Solar Cells (PQDSC) hold great promise for future renewable energy solutions. Utilizing Perovskite Quantum Dot Layers as the active layer in solar cells exploits quantum confinement, if the crystal size is below the Bohr radius [1], resulting in high power conversion efficiencies, a high photoluminescence quantum yield (PLQY)a narrow photoluminescence (PL) peak, and enhanced stability compared to bulk perovskite.[1] The versatility of X halides (I-, Br-, Cl-) and A cations (FA+, MA+, Cs+) allows precise bandgap control across the visible spectrum of the ABX3 perovskite structure.[2]
This study focuses on Cesium Lead Iodide (CsPbI) and Formamidinium Lead Iodide (FAPbI) perovskite quantum dot layers, exploring various washing processes in between varying PQD ratios in a mixed precursor solution. In-situ grazing incidence wide-angle scattering (GIWAXS) is used to reveal crystal structure and texture during thin-film formation. The potential integration of machine learning offers insights for optimizing PQD thin film fabrication in the future.[1] L. Liu et al., Adv. Sci. 9.7, 2104577 (2022)
[2] L. Protesescu et al. Phys. Nano. Lett. 15.6, 3692–3696 (2015)Speaker: Thomas Baier -
18:30
In situ study of morphology evolution of block copolymer templated metal oxide films 20m
Scalable production of the thin film is interesting for the commercialization of these materials. A fundamental understanding of the structure evolution during deposition is of great importance to tailoring the mesostructures. In a diblock copolymer-assisted sol-gel chemistry method, hybrid films of metallic species and polymer are formed with slot die coating. Pure block copolymers are deposited as control. In situ grazing-incidence small-angle X-ray scattering measurements are performed to investigate the self-assembly and co-assembly process during the film formation. A face-centered cubic (FCC) structure is preferentially formed in the hybrid film with improved order compared to the pure block copolymer thin films. A superlattice-like mesoporous metal oxide film is obtained by removing the polymer template from the hybrid films. The large data set of in situ GISAXS data will be used for machine learning applications.
Speaker: Guangjiu Pan (Technical University of Munich, TUM School of Natural Sciences, Department of Physics, Chair for Functional Materials) -
18:30
Information theory elements for data analysis 20m
Information theory serves as a practical tool for converting intuitive information into quantifiable numerical values. One key measure, Shannon entropy, plays a role in measuring information content within probability distribution functions. Similarly, mutual information proves valuable in determining correlations between variables, even if they are non-linear. Despite its potential, information theory has not been overlooked in the current landscape of machine learning. This presentation aims to address this gap by presenting relevant measurement metrics that could contribute to the development of machine learning-based approaches in the analysis of experimental data.
Speaker: Luis Carlos Pardo Soto (Universitat Politècnica de Catalunya) -
18:30
Injectable hydrogels from thermoresponsive tri- and tetrablock terpolymers investigated using scattering methods 20m
Lower critical solution temperature (LCST) polymers have attracted great interest for 3D bioprinting, as they can form a runny solution at room temperature, but a hydrogel at body temperature. In block copolymers featuring LCST blocks, the mechanical properties in the gel state strongly depend on the architecture of the polymer. Here we address an ABC triblock terpolymer and a BABC tetrablock terpolymer consisting of the hydrophilic OEGMA (A), the hydrophobic BuMA (B), and the thermoresponsive DEGMA (C).
The results from dynamic light scattering (DLS) on dilute solutions indicate that the hydrodynamic radii of the micelles formed by both, ABC and BABC, increase strongly above 25 °C, and the solutions feature a cloud point, i.e. aggregation of the micelles sets in. By synchrotron small-angle X-ray scattering and neutron scattering (SAXS/SANS), we found that the triblock terpolymers ABC form spherical core-shell micelles, that transform into cylinders at high temperatures, and then form compact large aggregates upon further heating. In contrast, the core-shell micelles formed by tetrablock terpolymers BABC stay spherical and form small aggregates at higher temperatures. Particularly, at high concentration, BABC transforms into an elastic two-compartment network upon further heating. Hence, the additional hydrophobic block in BABC results in a different type of gel formation.
Speaker: Feifei Zheng (Technische Universtität München) -
18:30
Integrating Machine Learning into X-ray Data Analysis: Insights from GRADES at SOLEIL 20m
In this paper, we present the GRADES team's exploration and implementation of machine learning (ML) techniques at the SOLEIL synchrotron radiation facility in Saclay. Our work encompasses three distinct use cases, each demonstrating the potential of ML to revolutionize data analysis in large-scale photon facilities.
Firstly, we detail the development of an X-ray diffractogram classification tool, which employs ML algorithms to quickly determine lattice types and space groups, thereby enabling faster and high throughput material analysis.
Secondly, we explore the application of large language models in creating an assistant app designed to streamline the management and utilization of SOLEIL’s extensive documentation stored in Confluence, JIRA, log-books, and our experiment databases. This tool aims to significantly improve accessibility and user interaction with critical data.
Lastly, we delve into the use of neural networks for the denoising of spectral images, specifically in the context of Angle-resolved photoemission spectroscopy (ARPES). Our approach demonstrates notable improvements in spectral image quality.
Collectively, these case studies highlight the versatility of ML in various aspects of synchrotron data analysis. We deliver our applications to all our users through our remote data treatment service "DARTS" [1,2].
[1] https://gitlab.com/soleil-data-treatment/soleil-software-projects/remote-desktop/
[2] https://doi.org/10.21105/joss.05562Speakers: Emmanuel FARHI (Synchrotron SOLEIL), Anass Bellachehab (Synchrotron SOLEIL) -
18:30
Investigation of detector backlighting and other effects in neutron darkfield images 20m
Neutron imaging can provide unique contrast mechanisms. In order to yield reliable and reproducible attenuation coefficients for quantification, one needs to fully understand and characterize the experimental setup. One effect that has been largely overlooked in scintillator-camera based neutron imaging systems, is the backlight scattering or back illumination in the detection system itself, which can significantly affect the quantification of attenuation coefficients and can lead to severe errors and image artifacts. Herein, the backlighting effects are investigated by varying the illuminated detector area and the magnitude of the attenuation. The attenuation coefficient of multiple metal plates was determined by polychromatic neutrons at the CONRAD V7 instrument. We found that the strength of the back illumination effect strongly depends on the sample absorption. While it is relatively moderate (a few percent) for weak absorbing samples, it can be severe when the sample is a strong absorber (or when it is comparable thick).
Speaker: Dr Ala'a Al-Falahat (Department of Mechanical Engineering, Faculty of Engineering, Mutah University, Al-Karak, Jordan) -
18:30
KDSource, an Open-Source Code for the generation of Monte Carlo particle sources using Kernel Density Estimation 20m
The design of neutron instruments usually is related to the calculation of radiation beams, and these simulations are normally decoupled from the source since the nuclear reactions that govern the generation of particles in the source are independent of the specific interactions that take place in the beam path. Also, radiation beams are usually transported far away from the source to reduce the background signal in the measurements and the radiation dose of the personnel. When evaluating the beam under different operating conditions, it is useful to have a source that can be re-sampled.
Different types of solutions are available to solve this problem: capture the particles that are produced in the position of the source and transport them into the beam, use track files with the particles that cross a specific surface and reuse them in a downstream simulation, or use a synthetic source, fitting an analytical distribution for each of the source variables (energy, position, direction and time) are recorded
KDSource [1] is an open-source code that uses the adaptive multivariate Kernel Density Estimation (KDE) method to estimate the source distribution at a given point in the beam trajectory, which seeks to overcome the discussed limitations of the previous approaches. This approach presents a novel methodology to optimize source modeling using adaptive multivariate kernel density estimation, which may be especially suited for radiation beam and radiation shielding simulations.
The core idea of the methodology is to use some machine learning libraries and algorithms (like scikit-learn in Python) to optimize the bandwidth selection for each source variable. With this strategy, smooth estimates of the variable distributions may be obtained from particle lists at a given point in a simulation that maintains correlations among variables. The code implements the proposed methodology in Python and C, and it consists of a module for KDE model optimization and another for sampling (i.e. generating new particles using the previously optimized model).
The objective of this work is to present to the community the theory behind kernel density estimation, and how the KDSource code works. Also, some examples of using this tool to simulate neutron time-of-flight experiments and to design the neutron imaging instruments for the High Brilliance Neutron Source project will be shown.
[1] N.S. Schmidt et al, 2022. KDSource, a tool for the generation of Monte Carlo particle sources using kernel density estimation. Ann. Nucl. Energy 177.
Paper URL: https://doi.org/10.1016/j.anucene.2022.109309
GitHub URL: https://github.com/KDSource/KDSourceSpeaker: Mr Norberto Schmidt (Forschungszentrum Jülich) -
18:30
KI4D4E: Machine Learning Approach for Digital Volume Correlation in 4D Computed Tomography Data at Synchrotron Radiation Beamlines 20m
The Helmholtz-Zentrum Hereon is operating imaging beamlines for X-ray tomography (P05 IBL, P07 HEMS) for academic and industrial users at the synchrotron radiation source PETRA III at DESY in Hamburg, Germany. The high X-ray flux density and coherence of synchrotron radiation enable high-resolution in situ/operando tomography experiments. Here, large amounts of 4D data are collected from a wide variety of samples, which is challenging to reconstruct, process, and analyze. In this multi-disciplinary project - KI4D4E, we utilize modern machine learning methods for the data processing of synchrotron-radiation tomography experiments, such as micro- and nano-CT simulation, denoising and artifact removal, phase retrieval, and digital volume correlation.
In this talk, we will present the methodologies and challenges to apply state-of-arts machine learning methods to digital volume correlation for the data analysis of biodegradable implant materials based on the high-resolution micro-CT datasets.Speaker: Tak Ming Wong (Helmholtz-Zentrum Hereon) -
18:30
Leveraging Automatic Differentiation in Complex Model Fitting 20m
Understanding laser-solid interactions is important for the development of laser-driven particle and photon sources, e.g., tumor therapy, astrophysics, and fusion. Currently, these interactions can only be modeled by simulations that need to be verified experimentally. Consequently, pump-probe experiments were conducted to examine the laser-plasma interaction that occurs when a high intensity laser hits a solid target. Since we aim for a femtosecond temporal and nanometer spatial resolution at European XFEL, we employ Small-Angle X-ray Scattering (SAXS) and Phase Contrast Imaging (PCI) that can each be approximated by an analytical propagator. In our reconstruction of the target, we employ a gradient descent algorithm that iteratively minimizes the error between experimental and synthetic patterns propagated from proposed target structures. By implementing the propagator in PyTorch we leverage the automatic differentiation capabilities, as well as the speed-up by computing the process on a GPU. We perform a scan of different initial parameters to find the global minimum, which is accelerated by batching multiple parallel reconstructions. The fully differentiable implementation of a forward function may serve as a physically-constraining loss, enabling training with unpaired data or unsupervised training of neural networks to predict the initial parameters for the gradient descent fit.
Speaker: Erik Thiessenhusen (HZDR) -
18:30
Machine Learning (ML)-Assisted Fabrication and Scattering data for Solar Cells 20m
Research on Machine Learning(ML) for Organic Solar Cells (OSCs) has currently tremendously increased. The performance of OSCs specifically depends on solvents, crystallinity, molecular orientation of absorbing layer, and morphology of active and interfacial layers. The complex nature of organics is demanding more efficient and eco-economic, and eco-friendly ML models such as photovoltaic phenomena are related to microscopic properties and require high-accuracy quantum calculations. For high accuracy, large-scale virtual screening is required, but on the other hand, high computational cost made it difficult for large-scale virtual screening. When a researcher fabricates a novel device from a novel material system, it often takes many weeks of experimental effort and data analysis to understand why any given device/material combination produces an efficient or poorly optimized cell. Here, we combine machine learning, device modelling and experimentation to effectively optimize the OPV fabrication process. ML techniques can effectively model the correlation between the properties of the OPV materials and the corresponding fabrication methods if they are trained using sufficient experimental data. Moreover, we highlight the integration of machine learning methods into the typical workflow of scattering experiments. We focus on scattering problems that faced challenge with traditional methods but addressable using machine learning, such as leveraging the knowledge of simple materials to model more complicated systems, learning with limited data or incomplete labels, identifying meaningful spectra and materials’ representations for learning tasks, mitigating spectral noise, and many others.
Speaker: Shuxian Xiong (DESY & TUM) -
18:30
Machine Learning Approaches on X-ray Scattering Beamline: First results and perspectives 20m
To face the ever growing massive and complex data collected on real materials during samples mapping and screening or in situ measurements at synchrotron beamline, fast software assistance with a minimum user input is nowadays required before, during and after the experiments.
On the french CRG IF BM32 beamline at the European Synchrotron (ESRF) X-ray scattering experiments are carried out for the study of surfaces and interfaces. Recently, we designed a Neural Network (NN) for the recognition of hkl Miller indices of Laue spots in Laue patterns produced by the microbeam illumination of polycrystals. During the training, the NN learned a very large number of possible configurations of Laue spot neighborhoods from simulated data. Compared to usual indexing technique applied to several crystals superimposed Laue patterns, we achieved a general speed up, even to a factor 100 in the low unit cell symmetry [1], allowing the online analysis of data.
In the framework of the french national DIADEM project aiming at the acceleration of materials design, the beamline is starting developments using machine learning approach to increase the throughput of the beamline. First we intend to build automatic systems for the beamline optics alignement and correction. Then we plan to optimize the acquisition by detecting region of interest and adapting the motors scan parameters (velocity, exposure, trajectory). Finally for the data analysis to determine the structural properties of the specimen, we focus on one hand on the automation of the inversion of the X-ray reflectivity spectra, and on the other hand the classification of Laue spots by their 2D shape and the recognition of possible corresponding extended defaults (dislocations).References:
[1] R.R.P. Purushottam Raj Purohit et al, J. Appl. Cryst. (2022). 55, 737–750
Speaker: Dr Jean-Sébastien MICHA (Univ. Grenoble Alpes, UMR SyMMES CNRS CEA/IRIG and CRG IF BM32 beamline at ESRF) -
18:30
Machine learning for the generation of virtual histology images from X-ray phase contrast tomography of biodegradable metal bone implants 20m
Histology remains the gold standard for the visualization and study of biological tissue in clinical pathology and biomedical research. However, the typical workflow entails time-consuming sample preparation steps whereby the tissue is first fixed, embedded and sectioned into slices before chemical staining, after which each slice is individually scanned by optical microscope. In comparison, synchrotron x-ray phase contrast (PC) tomography can be performed on the same subject material without the need for staining or sectioning, allowing for the simultaneous acquisition of data across the full sample volume that can be virtually sliced in any plane. This project examines the transformative potential of machine-learning techniques to combine the experimental efficiency of x-ray tomography with a synthesized image contrast that is characteristic of histological staining, a so-called virtual histology. At the Helmholtz-Zentrum Hereon (with research outstation based at the DESY campus in Hamburg, and beamlines and laboratories hosted by the Hereon Institute of Materials Physics), the Institute of Metallic Biomaterials is working on the study and development of biodegradable magnesium alloys as bone implant material. In those studies a suite of multi-modal imaging techniques over a wide range of scale have been utilized. For the training of our GAN neural network, over 30 data set pairs of both CT and then histology measurements performed on the same murine bone implant samples have been carefully co-registered through the development of our in-house 3D-2D multimodal registration software. Here we would like to present preliminary results of this project and discuss various aspects of the training requirements, our model assumptions and key pre-processing steps noted thus far.
Speaker: Sarah Irvine (Helmholtz-Zentrum hereon) -
18:30
Machine learning methods research on predicting protein profile parameters by synchrotron radiation small-angle X-ray scattering 20m
Synchrotron radiation light sources are widely used in various scientific fields due to their high performance and high energy. In the field of biology, protein function mechanism research is promoted by decoding protein structures and studying the correlation with their functions. Then the function mechanism advances scientific development in many fields, such as drug and new material research and development. The traditional method of X-ray diffraction experiments on crystalline protein samples makes it difficult to visually characterize the true structure of proteins in physiological states. The synchrotron radiation small-angle X-ray scattering experiment is convenient to perform for protein samples in the solution state. By analyzing the measured scattering intensity data, some low-resolution structural information of proteins can be obtained. Traditional software analysis methods usually fit a profile model which takes minutes to hours. We develop a machine learning analysis method to enable fast and accurate prediction of protein profile parameters, including molecular weight, maximum diameter, and radius of gyration, from scattering intensity data. We first create a labeled dataset of protein profile parameters and scattering intensities, including simulation data, experimental data from the literature, and data collected from small-angle X-ray scattering experiments. The dataset is preprocessed and then divided into three categories: training, validation and test. Next, we build the training framework for the supervised machine learning model and design the objective loss function between predicted and labeled profile parameters. Finally, the prediction model is trained and optimized by iteratively minimizing the value of the objective loss function. Thus, it is realized to directly and efficiently predict protein profile parameters from bulk scattering data. The prediction model can achieve second responses and improve the efficiency by a hundred to a thousand times, thus enabling real-time online high-throughput data parsing. The real-time feedback on the dynamics of protein profiles can be monitored during experimental processes with this prediction model. More in-depth research on protein function mechanisms can be promoted and provide the potential to extend scientific applications.
Speaker: Qingmeng LI -
18:30
Machine learning short-range spin correlations in pyrochlore antiferromagnet Gd2Hf2O7 20m
Discovering new phases of condensed matter with novel properties is of vital importance for fundamental and applicational research. Classical Monte Carlo simulations are commonly employed to study phases by stochastically sampling states and evaluating physical quantities from such states. Recently, machine learning has proven useful in classifying, identifying, or interpreting datasets from Monte Carlo simulations of classical spin models [1]. In this study, we utilized machine learning to analyze spin states in real materials, in conjunction with polarized neutron diffuse neutron scattering and reverse Monte Carlo refinements.
The pyrochlore antiferromagnet serves as a prototypical model for studying frustrated magnetism, offering a fertile ground for novel states where conventional long-range orders are generally suppressed [2]. We synthesized a candidate material, Gd2Hf2O7, and investigated its magnetic properties using macroscopic and neutron scattering measurement techniques. Although ordering and spin glass transitions were indicated by AC susceptibility, polarized neutron diffuse scattering revealed a liquid-like magnetic structure factor without any magnetic Bragg peaks. Reverse Monte Carlo refinement of the scattering pattern was employed to generate spin configurations that could reproduce the observed structure factor. Principal component analysis was found to successfully identify short-range Palmer-Chalker spin correlations in Gd2Hf2O7, as predicted by theorists [2].
References
[1] J. Carrasquilla and R. G. Melko, Nature Physics 13, 431 (2017).
[2] S. E. Palmer and J. T. Chalker, Physical Review B 62, 488 (2000).Speaker: Jianhui Xu (RWTH Aachen Uni., JCNS) -
18:30
Machine Learning-Based Analysis of X-ray Scattering for Characterizing Guadua Bamboo 20m
The primary objective of the study is to leverage machine learning methodologies to discern the contributions of various cell types within bamboo structure to the observed scattering patterns. This study employs a comprehensive dataset comprising 145 two-dimensional (2D) wide-angle x-ray scattering (WAXS) patterns obtained from a linear scan over a radial slice of a Guadua bamboo specimen, accompanied by a computed tomography (CT) imaging of the subvolume of the specimen studied with WAXS.
The first approach in this study involves mapping principal components extracted by principal component analysis (PCA) from the 2D WAXS patterns against the fiber ratio. The fiber ratio, derived from the CT reconstruction, is here the proportion of fibrous tissue relative to the total tissue (fiber and parenchyma) content within the bamboo cross-section. This quantitative measure serves to assess the composition of different tissue types in the bamboo structure. Subsequently, a random forest regression algorithm is employed to establish a correlation between these principal components and the fiber ratio. The resulting model is then utilized to generate representative scattering data for each distinct tissue type within the bamboo. To validate the results, the scattering patterns generated with the model are compared to the WAXS patterns best representing the different tissue types based on CT imaging.
The second approach is similar to the first, with the distinction lying in the use of a shallow neural network instead of a random forest algorithm. The first two principal components from the PCA are fed into the neural network to establish a mapping to the fiber ratio. The resultant model is then employed to generate representative scattering data for the various bamboo tissue types. These results are subsequently compared against the parenchyma scattering pattern to assess the effectiveness of the neural network approach.
The significance of this research lies in its innovative application of machine learning techniques to elucidate the distinct contributions of different tissue types in WAXS patterns from Guadua bamboo. It uses a multimodal data set and compares the outcomes of the random forest and neural network approaches. The study aims to determine the most effective method for discerning and representing bamboo tissue types based on x-ray scattering patterns, a methodology that could also be used for other heterogeneous materials. This approach not only enhances our understanding of bamboo microstructure but also contributes valuable insights into the broader field of material characterization using machine learning methods.Speaker: Enriqueta Noriega Benitez (Aalto University) -
18:30
Machine Learning-based Auto-indexing of Neutron Diffraction Pattern from China Spallation Neutron Source 20m
A neutron diffraction pattern auto-indexing algorithm based on machine learning was developed and customed for the diffraction pattern collected from China spallation neutron source (CSNS). Over three hundred thousand crystal structures with different symmetries from the Crystallography Open Database generate the neutron diffraction time-of-flight patterns. In addition, the background and instrument parameters from CSNS modulated the patterns to make them simulate the real-world patterns in CSNS as much as possible. The modulated patterns are the samples composing the training set and evaluation set. The real-world patterns collected from CSNS compose the test set. In this algorithm, the convolutional layers extract the symmetry-related features from samples and the afterward fully connected layers classify samples into different crystal systems and space groups. Based on the prediction result, the algorithm provides several options listed in descending order of likelihood. Apart from symmetry prediction, the algorithm also predicts the lattice parameters and the architecture is still the convolutional neural network. Due to variations in the number and composition of lattice parameters across different crystal systems, each crystal system owns a custom CNN architecture for parameter prediction. The auto-indexing algorithm will be integrated into the CSNS data reduction program.
Speaker: Rong Du (institute of high energy physics) -
18:30
Machine Learning-Based Crystal Structure Direct Prediction from Neutron Powder Diffraction 20m
The neutron powder diffraction data represent a one-dimensional projection of the three-dimensional structural information. Compared to single crystal neutron diffraction, the reduction in data dimension adds complexity to structural determination from neutron powder diffraction data. Structure determination with neutron powder diffraction is predominantly a manual endeavor, requiring intricate, specialized expertise to execute. Considering the powerful data dimension reduction and feature extraction capability of machine learning, a machine learning-based algorithm was developed to predict crystal structure directly from neutron diffraction pattern. Over three hundred thousand crystal structures with different symmetries from the Crystallography Open Database generate the neutron diffraction time-of-flight patterns. The crystal structures were coded to the coulomb matrix by the Ewald sum matrix representation and then composed the training set. The training set was used to train an auto-encoder compressing the coulomb matrix into a latent space. With the assistance of this well-trained auto-encoder, the neutron scattering pattern can be fitted by optimizing the latent space and the coulomb matrix and then the crystal structures can be reconstructed from the latent space. The optimization process is completed by the genetic algorithms.
Speaker: Hao Wang (China Spallation Neutron Source) -
18:30
Machine Learning-driven Quantification of XRF Data: Integrating Sample Generation and Simulation for Enhanced Analysis 20m
This poster presentation will introduce the development and application of machine learning techniques for elemental concentration quantification of X-ray Fluorescence (XRF) spectra collected by a laboratory setup and the surrounding techniques needed, with a focus on sample generation and simulation. XRF analysis is a powerful tool for elemental sample characterization, yet the accuracy, reliability and performance of analytical approaches will be influenced by complex sample composition and matrix effects.
Our approach involves the development and utilization of machine learning models for the quantification process in order to overcome challenges associated with traditional analytical methods. The poster will explain the design and implementation of these models, highlighting their capacity to adapt and learn from complex datasets.
A main part of our machine learning approach is the utilization of sample generation, ensuring diversity and the representativeness of the training dataset to the real world. By simulating a broad range of samples with varying elemental compositions, we aim to enhance the robustness and generalization ability of the machine learning model. This addresses directly the challenge of limited experimental data, allowing for more comprehensive and reliable model training.
Based on the sample generation we will discuss the simulation of XRF spectra. They serve as a essential component in training and refining our machine learning models. Additionally through the generation of synthetic datasets, we aim to assess the models performance under various experimental setup conditions, ensure their robustness and adaptability to real-world scenarios.
The presented work is still under progress but will finally contribute a significant tool for elemental analysis with XRF and will open up a path for broader applications of machine learning in XRF in order to overcome challenges associated with complex sample systems. The integration of machine learning, sample generation, and data simulation offers a comprehensive approach to enhance the robustness, accuracy, reliability and speed of XRF quantification.Speaker: Frank Förste (TU Berlin) -
18:30
Making photon and neutron open data machine-learning ready: a workshop and a roadmap 20m
Over the last decade, many European Photon and Neutron (PaN) facilities have adopted open data policies, making their data available for the benefit of the entire scientific community. This open data has a huge potential to be used for machine learning training, if and only if it is machine-accessible and FAIR.
To try and understand where we stand in the PaN community regarding the ML-readiness of our open data, we have organised a workshop in October 2023 at the SOLEIL synchrotron. This workshop included both sides of the table: data producers and data consumers.
In this presentation, we will present the status, challenges and opportunities identified during the workshop. We will also present the roadmap that emerged from these discussions, outlining a practical plan to improve our data policies, data and metadata quality checks, and current acknowledgment systems to be more tailored to ML applications.
Finally, we will highlight the potential impact of the roadmap on creating a more collaborative and efficient research environment where open data and ML work hand in hand.
Speaker: Peter Steinbach (Helmholtz-Zentrum Dresden-Rossendorf) -
18:30
Mapping the ICSD 20m
In this talk, I will discuss mapping the inorganic materials that have been reported in the ICSD [1]. This is important for both Materials Genome Initiative (MGI) [2] approaches to finding new materials and for adequately judging the uncertainty in machine learning approaches to structural determination from diffraction data.
We use a measure of structure similarity to determine how similar one crystal structure is to another. Given this similarity measure, we use community detection methods [3] and hierarchal clustering to find families of structurally similar materials. We demonstrate results from a small sampling of the ICSD. In the future, we will expand this to cover the entire database.[1] NIST Inorganic Crystal Structure Database, NIST Standard Reference Database Number 3, National Institute of Standards and Technology, Gaithersburg MD, 20899, DOI: https://doi.org/10.18434/M32147
[2] National Science and Technology Council, Materials Genome Initiative Strategic Plan (Nation-al Science and Technology Council, 2014), https://www.whitehouse.gov/sites/default/files/microsites/ostp/NSTC/mgi_strategic_plan_-_dec_2014.pdf.
[3] Community Detection in Graphs, Santo Fortunato, Physics Reports 486, 75 (2010). https://doi.org/10.1016/j.physrep.2009.11.002
Speaker: William Ratcliff (National Institute of Standards and Technology) -
18:30
McStas Union simulations of a 15 T magnet and background prediction with Machine Learning 20m
Neutron scattering allows for quite complicated sample environments with control over the sample conditions, such as temperature, as well as for the presence of strong magnetic fields. The presence of magnets in scattering experiments necessitates a significant amount of material in the structure. The coils of the magnets, outside the direct beam, add more material into the structure and could influence the experiments, since neutrons would scatter multiple times before reaching the detector. Additionally, they exert large magnetic forces on the structure that need to be withstood, requiring more material to safeguard the structural integrity of the system.
In an attempt to investigate the effect of the sample environment on the resulting background scattering, simulations of elastic neutron scattering data in the presence of multiple scattering from the sample environment were carried out. A model of the 15 T magnet for the BIFROST spectrometer at ESS was constructed with the Union tool in McStas, a neutron ray-trace simulation package. The contribution of the sample environment towards background was studied and analysed.
Furthermore, the model was parameterised to cover different experiment setups with a number simulation parameters, generating a substantial amount of simulation results. A comprehensive database of 24000 simulation results was constructed, analysed and utilised for the training, optimisation and testing of Machine Learning models that were able to predict the background of simulated experiments.
This novel approach can serve as an introduction to a new method of background recognition, paving the way for the development of automated background prediction tools that can be used within a wide range of instruments, with combinations of simulated and experimental data in the future.
Speaker: Petroula Karakosta (University of Copenhagen) -
18:30
Metainference simulations to interpret small-angle scattering experiments of non-conventional surfactants 20m
In the study of soft-matter systems, measurements performed in solution using, e.g., small-angle scattering are very important. Information on the size, shape, and dynamics of the system, can be obtained through modeling of small-angle neutron scattering (SANS) and small-angle x-ray scattering (SAXS) experiments. However, some systems can be challenging to model, due to non-conventional packing or polydispersion. In such cases, molecular dynamics (MD) simulations can help, but often the force fields do not reproduce the correct structural ensemble, or the events happen in a time scale longer than simulation times. Metainference is a Bayesian inference method that enhances the sampling of MD simulations through bias forces that drive the models towards improved agreement with the experiment. The goal is to sample configurations that represent the correct ensemble and, on average, obtain agreement with the experiment. Recently, some of us have extended Metainference to allow using SANS data. Here, we present the first study on surfactant aggregation combining SAXS and SANS Metainference MD simulations. We study Triton X-100, a detergent that has been previously studied in the literature, and for which there is no consensus on the formed micelles' size and shape. This is due to the non-conventional structure of the micelles, which cannot be described by a simple core-shell model, and polydispersion. A polydispersion of aggregates with sizes varying from 3 to 129 molecules is necessary to reproduce the SAXS and SANS spectra simultaneously. Triton X-100 micelles show shapes dependent on their size, with the smaller being rather spherical and the larger being irregular (oblate or triaxial shape). For some sizes, the hydrophobic part shows an onion-like structure. This case study illustrates how Metainference can aid the interpretation of small-angle scattering experiments.
Speaker: Henrique Musseli Cezar (University of Oslo) -
18:30
Neural Network based reflectometry analysis of liquid-gas interfaces 20m
Researchers from the University of Kiel and Tübingen, as part of the DAPHNE4NFDI initiative, are collaborating to enhance machine learning models for analyzing X-ray and neutron reflectivity datasets, exemplified by the Python package "mlreflect" [1]. This tool, developed at the University of Tübingen, utilizes artificial neural networks trained on solid sample reflectivity data, offering rapid predictions compared to traditional iterative least mean squares fitting methods. Successful experiments at the ESRF using a closed-loop system guided by machine learning data analysis demonstrated the potential of this approach [2].
The University of Kiel's expertise in X-ray reflectivity analysis of liquid samples ([3]) complements the mlreflect package through expanded and improved training data for different layer models in respect to liquid measurements. Recent experiments at ESRF on water-air interfaces validated the ML analysis pipeline at ID10. Based on the Maxwell Cluster a similar analysis pipeline at P08 could be implemented.
One challenge is the lack of sufficient experimental data, requiring reliance on simulated reflectivity data for training. Besides, metadata standardization is vital, with DAPHNE4NFDI developing a specialized schema, while the ORSO works on a file format for reduced reflectivity data. These efforts aim to provide essential metadata for analyses, ensuring consistency and accessibility for training machine learning models. Open reflectivity data examples, such as those shown by Linus Pithan on Zenodo, facilitate testing mlreflect prediction algorithms [4].
In summary, collaborative efforts are enhancing machine learning-driven analysis of X-ray and neutron reflectivity data. Challenges, including limited experimental data for test validation and metadata standardization, are being addressed, promising improved insights with the aid of open data resources and data aggregation platforms.
[1] Neural network analysis of neutron and X-ray reflectivity data: automated analysis using mlreflect, experimental and feature enginierring, A. Greco et al., Jounal of Applied Crystallography, 55, 362 (2022).
[2] Closing the loop: Autonomous experiments enabled by machine-learning-based online data analysis in synchrotron beamline environments, L. Pithan et al., J. Synchrotron Rad., 30(Pt 6), 1064–1075 (2023).
[3] A novel X-ray diffractometer for studies of liquid-liquid interfaces, B.M. Murphy et al., J. Synchrotron Rad., 21, 45 (2014).
[4] Reflectometry curves (XRR and NR) and corresponding fits for machine learning. L. Pithan et al., Zenodo (2022). https://doi.org/10.5281/zenodo.6497438Speaker: Svenja Hövelmann -
18:30
Online Classification of Diverse Metal Materials Using PGNAA and Machine Learning 20m
Recycling scrap as secondary raw material in Europe is not only the safest but also the most sustainable and economically viable source of raw materials. This option remains available despite political conflicts with mining countries. Moreover, engaging in recycling minimizes or avoids conflicts between the local population and the mining industry, particularly concerning human rights issues in those countries. Given the substantial and diverse mass flows in copper and aluminum production, there is a significant interest in real-time classification of recycling materials.
We present an approach that has enabled non-destructive online analysis of heterogeneous materials for the first time and is currently in use. This method relies on Prompt Gamma Neutron Activation Analysis (PGNAA), showing potential for non-destructive material analysis. The challenge in using PGNAA for online classification arises from limited and noisy data due to short-term measurements. Traditional evaluation methods, such as detailed peak-by-peak analysis, prove ineffective. To address the chalange, we suggest treating spectral data as probability distributions, enabling material classification through maximum log-likelihood.
For classification purposes, a fully measured spectrum is obtained for each material, and a kernel density estimator generates the corresponding probability distribution. Using the maximum (log-)likelihood method, unknown short-time measurements are assigned to materials based on the best-fitting distribution of a fully measured spectrum. This approach requires only a single fully measured spectrum for material classification, allowing for online classification without data preprocessing and additional training data.
The distribution can also be used to generate training, test, or validation data through sampling. This allows quick and easy generation of any number of spectra from a single source. Depending on the random sample size, simulation of short measurement times is flexible, eliminating the need for costly new data acquisition. Additionally, the generated data is crucial for parameter estimation of the kernel density estimator and the training of convolutional neural networks.
Experimental data includes 5 aluminum alloys, 5 copper alloys, and a total of 11 different materials (aluminum, cement, copper, E-scrap, stucco, soil, batteries, ore, melamine, PVC, and ASILIKOS). For pure aluminum alloys, near-perfect classification is achieved in under 0.25 seconds. To highlight the ease of classifying different materials, the measurement time is reduced to 0.0625 seconds, resulting in 100% correct classification.
Comparing our method with a Convolutional Neural Network (CNN), commonly used in spectrum classification, we demonstrate that our approach allows faster classification. Additionally, we employ Class Activation Maps (CAM) to visualize relevant spectrum areas during classification.
Speaker: Helmand Shayan (Deutsch) -
18:30
Operando GIWAXS Observation of the Stabled degradation process of Green-solvent Based PBDB-TF-T1: BTP-4F-12 Organic Solar Cells with EH-P as Solid Additive 20m
Solvent additives play an important role in organic solar cells. Traditional additives are mostly liquids, such as DIO, CN, DPE, which makes the solution fabrication process easy while, but they also share the disadvantage of being highly toxic. Thus, nowadays solid additives have called more research interest due to their various advantages in morphology-directing abilities, post treatment, enhanced device performance and stability. We explore an effective solid additive named EH-P in green-solvent based organic solar cells (PBDB-TF-T1: BTP-4F-12). A greatly increased device performance and stability are achieved with EH-P doping. In-situ GIWAXS and GISAXS are used to observe the evolution of micro-structure and crystallinity during the degradation process in air under illumination. The stability increasement mainly comes from morphology modification rather than photo-oxidation, which is proved with charge mobility measurements and UV-vis spectroscopy. The achieved scattering data will be treated with machine learning methods.
Speaker: ZERUI LI (TUM) -
18:30
Operando study on structure-activity relationship between electrolyte components and electrochemical performance for all-solid-state lithium-ion batteries 20m
All-solid-state lithium-ion batteries (ASSLIBs) have received extensive attention as one of the most promising power sources for flexible and wearable electronics. However, the practical application of ASSLBs has been hindered by poor interfacial stability and inferior ionic conductivity. Solid polymer electrolytes (SPEs) exhibit great potential in developing solid-state batteries, specifically for PEO and PEO-based derivatives, because of their superior interfacial compatibility, outstanding solubility against lithium salts, wide electrochemical windows, and high ionic conductivity. At the same time, solid fillers, as an important component in SPEs, play a crucial role in determining the overall electrochemical properties. Several strategies have been adopted to address the above issues, nevertheless, the SPEs degradation mechanism is still not clear and needs to be further studied. Therefore, we combined electrochemical characterization and morphological structure characterization to elucidate the structure-activity relationship between the component structure of the electrolyte and the electrochemical performance.
Speaker: Yingying Yan (Lehrstuhl für Funktionelle Materialien, Physik-Department, TU München) -
18:30
Phase behavior of thermo- and photoresponsive diblock copolymers for non-invasive schizophrenic switching 20m
Stimuli-responsive diblock copolymers (DBCPs) have gathered considerable interest for uptake, transport and release processes due to their property alteration upon exposure to external stimuli, such as temperature and light. In this study, DBCPs consisting of two thermoresponsive blocks, each with lower critical solution temperature (LCST) behavior and coil-to-globule transitions at the respective cloud points (CPs) are investigated in aqueous solutions. These are PNIPAM and azopyrazole (AzPy) functionalized PNDMAM. Upon exposure to UV light, the CP of the PNIPAM is expected to remain unchanged, while the CP of AzPy-PNDMAM may be tuned. This way, the DBCPs can be switched completely non-invasively and are expected to form unimers, (inverse) micelles, and aggregates in dependence on temperature. Here, we present the temperature-dependent phase behavior of a series of DBCPs with various block lengths and AzPy contents and in different isomeric states of the latter. Synchrotron small-angle X-ray scattering (SAXS) reveals that the DBCPs are expanded chains below the first CP and are collapsed at temperatures above, forming large aggregates without an intermediate micellar phase. Switching the photoactive group by UV irradiation does not have significant effect on this behavior.
Speaker: Peiran Zhang (Technical University of Munich, Department of Physics, Garching, Germany) -
18:30
Phase retrieval by a conditional Wavelet Flow: applications to near-field X-ray holography 20m
Phase retrieval is an ill-posed inverse problem with several applications in the fields of medical imaging and materials science. Conventional phase retrieval algorithms either simplify the problem by assuming certain object properties and optical propagation regimes or tuning a large number of free parameters. While the latter most often leads to good solutions for a wider application range, it is still a time-consuming process, even for experienced users. One way to circumvent this is by introducing a self-optimizing machine learning-based algorithm. Basing this on invertible networks such as normalising flows ensures good inversion, efficient sampling, and fast probability density estimation for large images and generally, complex-valued distributions. Here, complex wavefield datasets are trained and tested on a normalising flows-based machine learning model for phase retrieval called conditional Wavelet Flow (cWF) and benchmarked against other conventional algorithms and baseline models. The cWF algorithm adds a conditioning network on top of the Wavelet Flow algorithm that is able to model the conditional data distribution of high resolution images of up to 1024 x 1024 pixels, which was not possible in other flow-based models. Additionally, cWF takes advantage of the parallelized training of different image resolutions, allowing for more efficient and fast training of large datasets. The trained algorithm is then applied to X-ray holography data wherein fast and high-quality image reconstruction is made possible.
Speaker: Ritz Aguilar (Helmholtz-Zentrum Dresden-Rossendorf (HZDR)) -
18:30
Physics Informed Neural Networks for Neutron Transport in Large Sample Prompt-Gamma Activation Analysis 20m
Prompt-Gamma Activation Analysis (PGAA) measurement facilities for large samples have been intensively researched within the last years. Here, the interaction of the neutron flux field and the sample cannot be neglected. This leads to a nonlinear relation between the peak count rates and the elemental masses. Therefore, it is necessary to use an iterative evaluation procedure in this case. Within each iteration a full neutron transport simulation of the facility is required. Monte-Carlo methods enable accurate simulations of the neutron flux but they are also computationally expensive. Even though deterministic neutron transport calculations offer improved performance, the neutron transport simulations remain a computational bottleneck for the entire evaluation procedure. Neural Networks offer a promising alternative to classical methods. Once trained, the cost for executing a neural network is very low. In this talk, Physics Informed Neural Networks (PINNs) as solvers for the neutron transport equation are introduced. Instead of using large amounts of training data, these networks include physical information, in this case the neutron transport equation, in the training process. The potential benefits of the application of PINNs for large sample PGAA will be discussed, as well as future challenges.
Speaker: Alexander Jesser (Karlsruher Institut für Technologie) -
18:30
Providing datasets to characterize process- or deformation-induced structure formation of polymer materials via in situ synchrotron X-ray experiments 20m
In this contribution, an overview of experimental results obtained via simultaneous small- and wide-angle X-ray scattering (SWAXS) experiments is given to illustrate its importance in polymer science. Owing to the high spatial and temporal resolution, which can be beyond that of conventional material characterization methods, in situ synchrotron SWAXS experiments are suitable for investigating the structure formation of polymer materials during processing or deformation. For such purposes, the challenge is to develop and provide experimental setups useable for in situ experiments. Such efforts were realized during past long-term projects (LTP) at PETRA III, DESY (Hamburg, Germany). In the case of investigating process- or deformation-induced structure formation of polymer materials, large datasets with a huge amount of information in terms of scattering and metadata are generated. These labeled and well-organized datasets are obtained from various sources, e.g. detectors, motors, sensors etc., and therefore have different data formats, which are (mostly) synchronized. Thus, the achieved datasets are very suitable for polymer material characterization on several length scales – in the range of a few to hundreds of nanometers, e.g. from crystalline to super-molecular structures. By coupling SWAXS with other in situ characterization techniques, such as spectroscopy or digital image correlation, the datasets for material characterization can be further improved, however getting more and more complex. In this context, well-trained and reliable Machine Learning (ML) models could contribute to an even better understanding of the complex material-process-structure-properties relationship for polymers, which can be far beyond current restrictions.
Speaker: Anna Katharina Sambale (Leibniz-Institut für Polymerforschung Dresden e.V.) -
18:30
Providing datasets to characterize process- or deformation-induced structure formation of polymer materials via in situ synchrotron X-ray experiments 20m
In this contribution, an overview of experimental results obtained via simultaneous small- and wide-angle X-ray scattering (SWAXS) experiments is given to illustrate its importance in polymer science. Owing to the high spatial and temporal resolution, which can be beyond that of conventional material characterization methods, in situ synchrotron SWAXS experiments are suitable for investigating the structure formation of polymer materials during processing or deformation. For such purposes, the challenge is to develop and provide experimental setups useable for in situ experiments. Such efforts were realized during past long-term projects (LTP) at PETRA III, DESY (Hamburg, Germany). In the case of investigating process- or deformation-induced structure formation of polymer materials, large datasets with a huge amount of information in terms of scattering and metadata are generated. These labeled and well-organized datasets are obtained from various sources, e.g. detectors, motors, sensors etc., and therefore have different data formats, which are (mostly) synchronized. Thus, the achieved datasets are very suitable for polymer material characterization on several length scales – in the range of a few to hundreds of nanometers, e.g. from crystalline to super-molecular structures. By coupling SWAXS with other in situ characterization techniques, such as spectroscopy or digital image correlation, the datasets for material characterization can be further improved, however getting more and more complex. In this context, well-trained and reliable Machine Learning (ML) models could contribute to an even better understanding of the complex material-process-structure-properties relationship for polymers, which can be far beyond current restrictions.
Speaker: Eric Euchler (Leibniz-Institut für Polymerforschung (IPF), Dresden, Germany) -
18:30
Revealing the effect of solvent additive selectivity on the formation kinetics in printed active layers for organic solar cells 20m
Solvent additives have received tremendous attention in organic solar cells as an effective way to optimize morphology and phase separation. However, most research primarily focuses on solvent additives with superior solvation for non-fullerene acceptors (NFA) over polymer donors, such as 1-chloronaphthalen (1-CN) and 1, 8-diiodooctane (1,8-DIO). Few researches are related to solvent additives characterized by better solubility for polymer donors than NFA. Furthermore, the impact of solvent additives is mainly investigated in the case of films prepared via spin coating rather than slot-die coating, which exhibits distinct differences in the kinetics of film formation. Hence, the influence of solvent additive selectivity on the kinetics of active layer formation in the printed active layer remains unknown. In this study, we use PBDB-T-2F as the donor and BTP-C3-4F as the acceptor and introduce two distinct solvent additives, one with superior solubility for PBDB-T-2F compared to BTP-C3-4F, and another with inferior solubility for PBDB-T-2F. The drying process of the slot-die coated active layers with different solvent additives is studied by in situ UV-vis absorption spectra and in situ grazing incidence wide angle X-Ray scattering (GIWAXS). The achieved scattering data will be treated with machine learning methods.
Speaker: Jinsheng Zhang (TUM) -
18:30
ROADMAP: Reflectometry-driven Optimization And Discovery of Membrane Active Peptides 20m
The COVID-19 pandemic underscores the urgent need for swift advancements in therapeutic discovery against emerging health threats. Membrane-active peptides (MAPs) are a class of bioactive compounds with diverse applications in antimicrobial activity and drug delivery across cell membranes. Despite their immense potential, the sheer complexity of the space of possible MAPs presents challenges in discovery efforts. Understanding the structure-function relationship of MAPs is pivotal, yet remains incompletely elucidated.
In response to these challenges, we initiated the ROADMAP project to establish a robust measurement framework for membrane-associated MAPs. The project involves the complete automation of neutron reflectometry (NR) measurements and sample preparation at the CHRNS CANDOR reflectometer, NCNR. The approach accelerates measurement times through experimental optimization using information theory before and active learning during data acquisition. Predictive ML models, derived from sequentially collected data, guide efficient future measurements, facilitating rapid refinement of the structure-function relationship.
The ultimate objective of ROADMAP is to curate an extensive NR dataset encompassing over 1000 MAP sequences. Our approach emphasizes autonomous experimentation and model building, demonstrating the ability to derive meaningful insights from sparse data. By bridging the gap between theory and experimentation, ROADMAP represents a pioneering effort in the quest for novel therapeutics with potential implications for combating infectious diseases and addressing public health challenges.
Speaker: Frank Heinrich (Carnegie Mellon University and NIST Center for Neutron Research) -
18:30
Strategy to Simulate and Fit 2D GISAXS Patterns of Nanostructured Thin Films 20m
Grazing-incidence small-angle X-ray scattering (GISAXS) is a widely used method for the characterization of the nanostructure of supported thin films and enables time-resolved in situ measurements. The two-dimensional (2D) scattering patterns contain detailed information about the nanostructure within the film and at its surface. Efficient and fast model fitting is often hampered because it is time-consuming to analyze the 2D patterns. Moreover, the structural information is not only distorted by the reflection of the X-ray beam at the substrate-film interface and its refraction at the film surface, but also by scattering of the substrate, the sample holder and other types of parasitic background scattering. In this work, a new, efficient strategy to simulate and fit 2D GISAXS patterns, that explicitly includes these effects, is presented and demonstrated at the example of (i) a model case nanostructured thin film on a substrate and (ii) experimental data from a microphase-separated block copolymer thin film [1]. To make the protocol efficient, characteristic line cuts through the 2D GISAXS patterns, where the different contributions dominate, are analyzed. The contributions of the substrate and the parasitic background scattering—which ideally are measured separately—are determined first and are used in the analysis of the 2D GISAXS patterns of the nanostructured, supported film. The nanostructures at the film surface and within the film are added step by step to the real-space model of the simulation, and their structural parameters are determined by minimizing the difference between simulated and experimental scattering patterns in the selected line cuts. While, in the present work, the strategy is adapted for and tested with BornAgain, it can be easily used with other types of simulation software. The strategy is also applicable to grazing-incidence small-angle neutron scattering.
- F. Jung, C. M. Papadakis, J. Applied Crystallogr. 2023, 56, 1330.
Speaker: Christine M. Papadakis (Technical University of Munich, TUM School of Natural Sciences) -
18:30
Supervised Machine Learning Approach for Phase Identification in Neutron and X-ray Powder Diffraction Experiments 20m
X-ray and neutron powder diffraction are experimental techniques that allow the
determination of structural properties of materials, such as their phase compo-
sition (identification and quantification). Machine learning has the potential
to efficiently replace the traditional procedural paradigm in phase determina-
tion due to its ability to learn data patterns and use them in predictions. In
this study, known structures of different phases were obtained from the Crys-
tallography Open Database and the corresponding powder diffraction patterns
were calculated. Systematic differences between the measured and calculated
diffraction patterns were analysed. A machine learning algorithm was trained
and benchmarked against measured data.Speaker: Loubna Kadri -
18:30
Tackling Laue pattern solving using neural networks 20m
Traditionally, the analysis of Laue diffraction pattern, crucial for determining the crystal orientation, has been a time-consuming process, requiring manual input of a skilled user. The development of an fully autonomous recognition tools aims to streamline this procedure, enhance accuracy, and to enable automation of various tasks such as crystal coalignment [1].
Existing Laue orienting software (for example OrientExpress, QLaue [2], LauePt [3] or LaueTools [4]) requires manual input and cannot solve Laue patterns automatically. In the recent years, problem is approached via machine learning. A paper by Purohit, et al. (LaueNN [5]) exlores a use of a preceptron architecture. Another possibility is the use of reinforced learning. On the other hand, images form x-ray detector itself could be directly processed using convolutional networks or generative models. The spatial correlation of data, the reflection spots, suggests a potential use of graph convolutional networks. We will discuss all these approaches and show our proposals for general problem of automatic Laue pattern solving.
[1] see contribnution "ALSA: Automatic Laue Sample Aligner", https://mambaproject.cz/alsa
[2] https://github.com/stuwilkins/QLaue
[3] https://github.com/yaafeiliu/LauePt4
[4] https://gitlab.esrf.fr/micha/lauetools/
[5] Purushottam Raj Purohit, Ravi Raj Purohit, et al., Journal of Applied Crystallography 55.4 (2022).Speaker: Tomáš Červeň (Charles University) -
18:30
The effect of a sputter-deposited TiOx interface modification layer on perovskite solar cells 20m
Efficiently suppressing non-radiative recombination within the hole-blocking layer (HBL) and at the HBL-active layer interface is critical for enhancing solar cell performance. In this study, the TiO$_x$ layer is sputter-deposited onto a SnO$_2$ layer at room temperature as a buried interface modification layer. We investigate the structural evolution of TiO$_x$ during sputter deposition using in situ grazing-incidence small-angle X-ray scattering (GISAXS). The novel HBL, achieved by depositing TiO$_x$ with an appropriate thickness on the SnO$_2$ layer, exhibits favorable characteristics, including suitable transmittance, smoother surface roughness, and reduced surface defects. Consequently, this leads to diminished trap-assisted recombination at the interface between the HBL and the active layer. The incorporation of the TiO$_x$ buried interface modification layer results in perovskite solar cells with enhanced power conversion efficiencies and stability compared to unmodified SnO$_2$ monolayer devices. The large data set of in situ GISAXS data will be used for machine learning applications.
Speaker: Xiongzhuo Jiang (Technical University of Munich, TUM School of Natural Sciences, Department of Physics) -
18:30
The small angle neutron scattering instrument KWS1 20m
Small angle scattering (SAS) is a widely used tool to address the nano-scale. It can be used for soft matter science, i.e. colloids, complex fluids, polymers, nanocomposites, proteins and protein complexes, and finally also in food science. But also in the field of materials, f.i. steels and alloys, it can be useful. When using polarized neutrons with/without polarization analysis, even more information can be obtained for steels and other magnetic materials. Finally, the grazing incidence geometry reveals detailed information about near-surface structures and hidden layers, be it non-magnetic or magnetic.
There are many attempts to support the data analysis and the interpretation using artificial intelligence (AI). This may lead to the choice of a correct theoretical model that then may be fitted using Bayesian statistics to obtain a statistically relevant statement about the original sample system. Also the scanning of a given parameter space (concentration, temperature, pressure, or other external fields) may support the quick parsing of a phase-diagram-like map without too many useless datapoints in the middle of an already characterized 'phase'. Basically, there are no limits to applications of AI combined with SAS.
In this contribution I present the instrument KWS1 with many technical details and a few scientific examples. The application of AI may be discussed by the interested audience and the presenter.Speaker: Henrich Frielinghaus (JCNS) -
18:30
The use of artificial neural networks for the unfolding procedures in neutron activation measurements 20m
A method called NAXSUN was developed to measure the effective neutron cross-sections of induced nuclear reactions [1,2,3]. It is based on irradiating multiple samples with energy-wide neutron fluxes and measuring the saturation activity using gamma spectroscopy. Cross section values are then obtained using unfolding techniques. So far, we have used SANDII, GRAVEL and MAXED algorithms for that purpose [4,5,6]. In this work, we used artificial neural networks for the unfolding procedure. Here we will present the obtained results, which were tested on the example of measurement of neutron-induced reactions on indium.
[1] N. Jovancevic, L. Daraban, and S. Oberstedt, Nuclear Instruments and Methods in Physics Research Section A:Accelerators, Spectrometers, Detectors and Associated Equipment 739, 68 (2014).
[2] N. Jovančević, L. Daraban, H. Stroh, S. Oberstedt, M. Hult, C. Bonaldi, W. Geerts, F.-J. Hambsch, G. Lutter, G. Marissens, et al., The European Physical Journal A 52, 148 (2016).
[3] S. Ilić, N. Jovančević, L. Daraban, H. Stroh, S. Oberstedt, M. Hult, C. Bonaldi, W. Geerts, F.-J. Hambsch, G. Lutter, G. Marissens, M. Vidali, and D. Knežević, The European Physical Journal A 56, 202 (2020).
[4] M. Reginatto and P. Goldhagen, Health physics 77, 579 (1999)
[5] W. N. McElroy, S.Berg, and T. Crockett, Los Alamos National Laboratory report, AFWL-TR-67-41 I-IV (1967).
[6] M. Matzke, Report PTB-N-19 I-IV (1994)Speaker: Nikola Jovancevic (University of Novi Sad) -
18:30
Tuning the bandgap of double perovskite by anion exchange 20m
Double perovskites, a relatively new kind of lead-free perovskite materials, have emerged with compelling characteristics, including low toxicity, extended carrier lifetime, and a small effective carrier mass. These unique attributes make them promising material for photovoltaic applications and draw considerable research interest. Among bismuth-based double perovskites, Cs2AgBiX6 (where X can be Cl, Br, or I) has stood out for its potential in photovoltaic applications, primarily attributed to its suitable bandgap. Some researchers studied thermoelectric properties of double halide perovskite Cs2AgBiI6 and proved that it is an excellent candidate for thermoelectric applications. They also investigated that Cs2AgBiI6 nanocrystals possess narrower bandgap than other halide Cs2AgBiX6. Thus, increasing the content of iodide ions in Cs2AgBiX6 can effectively narrow the band gap. In this work, the iodide ions will be introduced to Cs2AgBiBr6 by adding TMSI when spin-coating. It is reported that no more anion exchange happened with more TMSI. Therefore, Cs2AgBi(BrxI1-x)6 perovskite solar cells will be fabricated by solution method. The anion exchange process will be studied in this work. Morphology, device characterizations techniques like XRD, SEM, J-V curves, EQE spectra etc. would be taken to help optimize the morphology of thin films and PCE of solar cells. In-operando studies will be taken to identify morphology changes during device operation.
Speaker: Zhaonan Jin -
18:30
Using machine learning to optimize the crystal volume of protein crystals 20m
Modern chemical industry companies now routinely use machine learning to optimize protein-enzymes with respect to their yield in their enzymatic reactions. Those reactions can lead to the synthesis of complex drugs which would have to be produced in a more cost-intensive way without protein-enzymes as catalysts. Also, these optimized enzymes help to reduce the production of unwanted side products in these synthesis routes. The variation parameters are the amino acid sequence and the buffer conditions. The data base which the machine learning algorithm is based on is often produced by try and error methods in wet labs or relies on published structures found in the protein data base. In this contribution I propose to use these approaches to modify the outer surface of proteins in order to optimize their crystallization behavior to yield large volume crystals. Those crystals are needed for neutron diffraction. The resulting neutron structures resulting from this technique will lead to a better understanding of the enzymatic mechanisms of the respective enzymes. It will also elucidate the optimization process of the machine learning algorithms mentioned above. I propose to use alpha-fold to predict the fold of these newly designed proteins and I discuss the use of the protein data base as input for the optimization of the surfaces of these proteins for an optimal crystal growth.
Speaker: Tobias Schrader -
18:30
Using PyDevice to connect Beamline simulations to a controls framework (Bluesky/EPICS) 20m
Experimental Physics and Industrial Control System (EPICS) is a framework for developing distributed control systems. One of the modules available to EPICS is PyDevice which allows connecting python code to the process variables distributed by the EPICS control system. Bluesky is a higher level, user-facing framework for specifying the logic of experiments. In this poster, PyDevice will be used to connect simulated x-ray beam lines to the EPICS control system, creating “realistic” controls environment in which to test Bluesky-based experimental plans (e.g. using genetic algorithm or reinforcement learning).
Speaker: Max Wyman (Argonne National Laboratory) -
18:30
Validating the use of Gaussian Process Regression for Adaptive Mapping of Residual Stress Fields 20m
Neutron residual stress mapping is a valuable tool for determining the bulk residual stress state of large-scale engineering components. Probing the stress state using a high density of measurement points is time intensive and presents a limitation for what is experimentally feasible. These data are traditionally obtained using a brute force approach where data are measured for a discreet grid of locations. An active learning approach such as, Gaussian process regression (GPR), can incorporate information about previous measurements to achieve higher fidelity stress/strain maps by reconstructing individual fields from a subset of measurement locations. Results presented in this paper evidence that determining stresses from reconstruction strain fields is a viable approach for reducing the number of measurements needed to fully sample a component's stress state. Effects of errors in individual GP reconstructed strain maps and how these errors propagate to the final stress maps were assessed. Implications of the initial sampling approach and how localized strains affect convergence are explored to give guidance on how best to implement a dynamic sampling experiment.
Speaker: Chris Fancher (Oak Ridge National Laboratory) -
18:30
Versatile Inverse Problem Framework 20m
In the exploration of universe and matter, dealing with inverse problems is often a central challenge. In many experimental investigations, which are carried out in particular at large-scale research facilities such as FRM II, DESY or European XFEL, the essential phase information in the experimental data is lost due to the measurement principle (phase problem). Therefore, methods based on direct inversion are not applicable, so that the solution of the underlying non-convex optimization problem is usually very time-consuming and expensive to implement.
Here we present our project “Versatile Inverse Problem fRamework” (VIPR), recently funded by the Federal Ministry of Education and Research (Grant 05D23CJ1). The stated goal of our project is to develop a flexible software framework for data-driven solutions to inverse problems. First we plan to focus on using invertible neural networks. Other architectures can be also considered at later stages of the project. The main application areas envisioned include grazing incidence small- and wide-angle scattering with both neutrons and x-rays, neutron/x-ray reflectivity, and ptychography. Development will also take into account requirements from spectroscopy and particle physics.
Speakers: Dr Stefan Häusler (Forschungszentrum Jülich GmbH, JCNS), Egor Vezhlev (Forschungszentrum Jülich GmbH, JCNS), Dr Marina Ganeva (Forschungszentrum Jülich GmbH, JCNS)
-
16:50
-
19:00
→
23:00
Conference Dinner at Gasthof Neuwirt, Garching 4h Gasthof Neuwirt
Gasthof Neuwirt
Münchener Str. 10, 85748 Garching bei München
-
09:00
→
10:30
-
-
09:00
→
13:00
FRM II Tours: FRM II Tours: morning slots UBA 03 33 - Seminar room (MLZ)
-
09:00
→
13:00
Workshops 1: Analyzing Large Grazing-Incidence X-Ray Scattering Datasets Using INSIGHT UYM 03 30 - Meeting room (Geb. Ost) (MLZ)
-
09:00
Analyzing Large Grazing-Incidence X-Ray Scattering Datasets Using INSIGHT 4h
INSIGHT is a Python-based software tool for processing and analyzing grazing-incidence wide- and small-angle X-ray scattering data (GIWAXS/GISAXS) for large datasets (https://doi.org/10.1107/S1600576723011159). It focuses on efficient data management, customized scripting, and performant processing, and could be extended to ML approaches. The one-step software solution aims to accelerate the analysis of complex datasets of kinetic processes that shed light on the dynamic nano-assembly and structural evolution during in situ and operando studies. The introduction demonstrates basic functionalities and the general workflow in INSIGHT.
Speaker: Lennart Reb (TUM E13)
-
09:00
-
10:00
→
13:00
Workshop 2: DARTS: The Data Analysis Remote Treatment Service: AI demo UYW 04 44 - Seminar room (MLZ)
-
10:00
DARTS: The Data Analysis Remote Treatment Service: AI demo 3h
We present the Data Analysis Remote Treatment Service (DARTS) [1,2], an open-source remote desktop service that launches on-demand virtual machines in the cloud, and displays them in a browser. The released environments can be used for scientific data treatment, for example.
DARTS can be deployed and configured within minutes on a server, and can run any virtual machine. The service is fully configurable, supports GPU allocation, is scalable and resilient within a farm of servers. DARTS is designed around simplicity and efficiency. It targets laboratories and facilities that wish to quickly deploy remote data analysis solutions without investing in complex hypervisor infrastructures. DARTS is operated at Synchrotron SOLEIL, France, in order to provide a ready-to-use data treatment service for X-ray experiments.
In the scope of this conference, we shall demonstrate the applicability of the service to provide an AI-centric environment with GPU capability.
[1] https://gitlab.com/soleil-data-treatment/soleil-software-projects/remote-desktop/
[2] https://doi.org/10.21105/joss.05562Speaker: Emmanuel FARHI (Synchrotron SOLEIL)
-
10:00
-
10:15
→
13:00
Workshop 3: Machine Learning Basics UYG 02 22 - Pforte (MLZ)
-
10:15
Machine Learning Basics I 2h 45m
We are happy to invite you to our half-day satellite workshop "Machine Learning Basics" on Wednesday, April 10th in Garching at the MLZ.
The workshop is designed for participants keen on exploring basic machine learning techniques and their application to neutron data.
Throughout the workshop, you'll gain insights into fundamental concepts, discover practical applications, and apply your newfound knowledge through hands-on exercises. Finally, we provide you an opportunity to get in touch with one of the hot topics in the field of AI. This MLZ event is jointly organized by Data Evaluation group (DEVA) and JCNS Neutron SimLab.
Speakers: Marina Ganeva (JCNS at MLZ, Forschungszentrum Jülich GmbH), Dr Neelima Paul (Technical University of Munich, Heinz Maier-Leibnitz Zentrum (MLZ)), Stefan Häusler (Forschungszentrum Jülich GmbH, JCNS)
-
10:15
-
13:00
→
13:30
Lunch for workshop participants 30m MLZ
MLZ
-
13:30
→
17:30
FRM II Tours: Afternoon tours UBA 03 33 - Seminar room (MLZ)
-
13:30
→
16:00
Workshop 3: Machine Learning basics: part II UYG 02 22 - Pforte (MLZ)
-
13:30
Machine Learning Basics II 2h 30m
We are happy to invite you to our half-day satellite workshop "Machine Learning Basics" on Wednesday, April 10th in Garching at the MLZ.
The workshop is designed for participants keen on exploring basic machine learning techniques and their application to neutron data.
Throughout the workshop, you'll gain insights into fundamental concepts, discover practical applications, and apply your newfound knowledge through hands-on exercises. Finally, we provide you an opportunity to get in touch with one of the hot topics in the field of AI. This MLZ event is jointly organized by Data Evaluation group (DEVA) and JCNS Neutron SimLab.
Speakers: Marina Ganeva (JCNS at MLZ, Forschungszentrum Jülich GmbH), Dr Neelima Paul (Technical University of Munich, Heinz Maier-Leibnitz Zentrum (MLZ)), Stefan Häusler (Forschungszentrum Jülich GmbH, JCNS)
-
13:30
-
13:30
→
16:00
Workshop 4: spear_tools: Deep learning allrounder for free-electron-laser pulse characterization UYL 03 22a/b - JCNS Meeting room (MLZ)
-
13:30
spear_tools: Deep learning allrounder for free-electron-laser pulse characterization 2h 30m
The ultrashort and ultraintense pulses produced by X-ray free-electron lasers (XFELs) realize exposure times that typically lie in the femto- or even attosecond range. One of the long-term goals at free-electron lasers is to develop a diagnostic tool able to characterize the elusive temporal profile of these pulses in real-time and thus open new fields of atto-science with X-rays. In a practical regard, such an opportunity would also accelerate the progress during experimental campaigns as well as the data analysis afterward. We propose spear_tools – a framework suitable to do so using deep learning algorithms for the angular-streaking methodology, which emerged as a non-destructive technique able to retrieve the time-energy structure of XFEL pulses on a single-shot basis with attosecond resolution.
Currently, the framework includes these functionalities:
• Several simulation data generators necessary for neural network training
• A neural network development platform, including progress visualization
• Evaluation pipelines suitable for real-time and post-beamtime usage
• Visualization dashboards that can be used during and after experimental campaigns
spear_tools is suitable for beginners and advanced programmers, as only configuration files need to be changed or Jupyter Notebooks launched to get started. With its modular structure, spear_tools offers the option of quickly integrating custom simulation environments as well as neural network architectures and, beyond that, the extension to use cases other than the one presented here. Currently, the framework is only working in the simulation environment, but continuous developmental steps are taken to bring the analysis also to experimental data.Speakers: Kristina Dingel (Intelligent Embedded Systems, University of Kassel), Mr Thorsten Otto (Deutsches Elektronen-Synchrotron DESY)
-
13:30
-
09:00
→
13:00